Introduction
There have been many interactions about the promise of how generative AI and machine learning will revolutionize the way we conduct business. There is always a promise of what could be the next big thing on the scale of the “internet”. The market research industry, too, has grown by leaps and bounds. We are moving towards technology-reliant insights collection and management that scratches beneath the surface and showcases trends faster. Conversational AI has set us on the path of Research 3.0.
What is Research 3.0? In the words of Ray Poynter and NewMR, a QuestionPro partner, Research 3.0 is the link between things like chatbots, video, extended media, and observational research with non-experts.
The article discusses the use of the AI language model ChatGPT in surveys and the potential impact it may have on data quality and the research industry. Jamin, one of the commentators, believes that ChatGPT can revolutionize the way customer insights are gathered and analyzed by enabling natural conversation and analyzing open-ended questions with more sophistication.
The other panelists of this paper, Jamin Brazil and Vivek Bhaskaran, discuss how technology like ChatGPT can help with qualitative research, allowing for more efficient and accurate data processing and the opportunity to decouple from the survey in some cases. They also mention potential concerns around the impact of such technology on jobs traditionally part of the back-end field operations and research industry.
QuestionPro has implemented a system to detect when ChatGPT is used in open-ended responses and flag them for review. The text also mentions a separate project run by QuestionPro that helps non-researchers design surveys using ChatGPT to generate a list of questions around a particular topic.
- Introduction
- What is ChatGPT?
- The impact of ChatGPT in data gathering and data analysis in 2023
- The impact of ChatGPT on market research and what QuestionPro is doing about it
- What is the impact of digitization and ChatGPT on researchers?
- Does generative AI impact search-based taxonomy for knowledge management platforms?
- Negative implications of ChatGPT
- Is ChatGPT a threat to traditional market research or a superpower?
- Does generative AI impact panel and research quality?
What is ChatGPT?
ChatGPT is a generative AI engine with a chat interface where you can ask any questions, and it’ll come back with intelligent answers. Assistant-like features are among its functionalities; you can ask it to do things, create content and summarize it.
The platform works on the model of conservational AI and constantly trains itself to adapt and learn the basis of the persona you give to it. It can continuously evolve and learn from various parameters to be highly adaptive based on the user’s prompts.
It can be used to summarize text, answer questions, make iterative judgments, hypothesize and synthesize text in data, troubleshoot, and more. The possibilities are limitless!
ChatGPT or GPT-3 in the form currently released by OpenAI 175 billion parameters. However, GPT-4, which will soon replace the earlier model, will have 100 trillion parameters. Let that sink in for a second. The processing that is currently mind-boggling is going to pale in comparison when the new version launches.
Nothing defines ChatGPT better than asking itself, though; this is what it says about itself – ChatGPT is an extensive language model developed by OpenAI that is trained on a massive dataset of conversational text. It is designed to generate human-like text in response to a given prompt. It can be used for various natural language processing tasks such as language translation, question answering, and text summarization.
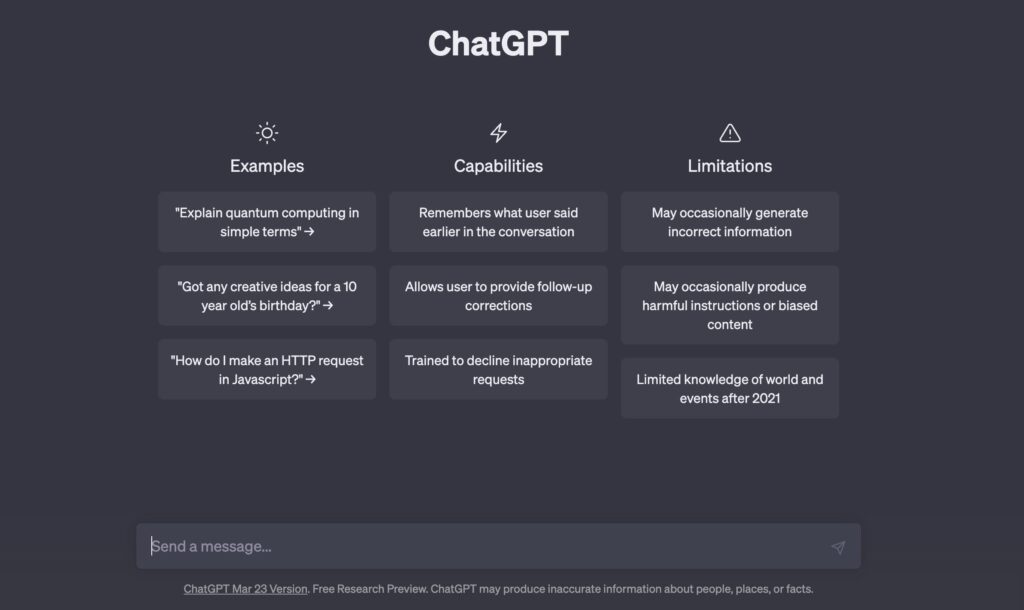
ChatGPT will significantly impact how we gather and analyze consumer data in 2023 more than the internet did from 1996 to 2006.
The impact of ChatGPT in data gathering and data analysis in 2023
You think about the advantage that the internet provides consumer insights professionals predominantly around speed. You could argue price, maybe, but I think the most significant benefit has been speed and accessibility. Before, we had to intercept people in malls to get them to complete a survey. Now we intercept them in their inboxes to get the same thing done, and certainly, in the period you referenced in the early 2000s. Online surveys were copied and pasted from phone inner phone interviews or model intercepts, so there wasn’t anything unique about it except for the format, the place it was being done, you fast forward to, let’s say, yesterday like pre-ChatGPT. You look at our industry; we are dealing with similar problems that we dealt with 20 or 30 years ago.
Unstructured data is one of the most significant wastes in consumer insights.
In the past, it has happened that brands conducting a customer sat survey would get about half a billion completes a year, so a lot of completes. And if they are majorly open-ended questions, that’s a lot of data to churn. Frequently, such brands would randomly select a segment of about 20 responses, hand-code those and say these are relatively representative of the sentiment on the subject in research. This model wasn’t foolproof.
If you start decoupling the framework, you think about the rationale of the actual survey, and its instrument is quite literally just a conversation at a scale.
Nobody speaks in Likert Scales; we don’t talk about; a five-point scale around how much you like your coffee. Instead, we have a conversation. This technology employs the same logic of enabling conversations to surface feelings and vectors and generate a highlight reel for us that is very actionable.
Research at Scale
The internet enabled quantitative research due to the survey angle, but qualitative research has primarily been labor intensive. The only reason qualitative research struggled in comparison is that it lacked the back-end technology and the ability to analyze at scale. ChatGPT is changing that paradigm for research.
GreenBook Research Industry Trends (GRIT) Report has been working with a text analytics provider called Yabble, which has a text analytics component that you know is relatively standard to get to themes and clustering. Leonard Murphy at GreenBookbut mentioned that when they used ChatGPT, he and the team were blown away at how the technology could interrogate the data to ask for a summary. GPT-3 wrote an excellent overview, even if it didn’t cover everything needed.
In a podcast that Lenny did with Simon Chadwick, they used the tool to summarize the discussion. It wasn’t full of wonderful flowy language, but it was accurate, summarized the salient points, and communicated skillfully and professionally. It did an excellent job of citing examples from the open-ended discussion and filling in the blanks. There was an ability to decouple from the survey in a few instances, but that was not a deal-breaker.
We have an opportunity; if we combine ChatGPT with other an existing technology stack that we’ve seen in use, like text analysts that get into emotional effect right, not just sentiment but actually to try and understand the emotion and the states of mind that are occurring within that textual conversation, voice analytics all of those things, there is a real opportunity to unlock immense value in discussions whether it’s via text or voice.
Big organizations that do a lot of insights collection and management can tap into this and deploy it at scale. Clients working with several models on the Gen 2 side are working with large-scale research buyers on the future of their insights organizations and developing the ability to unlock qualitative insights at the scale of unstructured data regardless of the form factor. It doesn’t matter whether it’s video or text, or voice; this is a huge priority for them. They want to be able to deploy this because they see the value of understanding people at a deeper level than what a survey can deliver, so it’s exciting times.
It’s also scary; let’s be honest, this could replace researchers from a process standpoint. I don’t think it’s anywhere close to being able to replace the thinking and the overall analysis. Still, it can streamline many jobs for people who have traditionally been part of the back-end field operations and research industry.
Summarization of insights
Another critical use case of ChatGPT is the ability to summarize information. We spoke about this briefly in the past, but it is one of the most significant use cases of the generative AI model. With a little bit of coercing and assigning a persona, you can get to a level of surfacing important information.
For example, from a productivity perspective, when you want to summarize something, you have to read an entire blog and then summarize it or read a whole set of reports and summarize it, which is not super intellectual. With, ChatGPT, you can summarize a complete transcript or an entire blog in five points if that’s what you need, which is a true game changer.
Many people in technology and software development believe we no longer need software developers because AI will write software, which is entirely untrue. In the practical, real world, you cannot mock up what a ScreenFlow should do, and then you got code that, you know, yes, there are code assists right now, same thing like summarization. They’re called assist tools. Having said that, if you write the first three words, it’ll write out the entire component. That’s how I think this AI will evolve around an assistant type of model; when you’re trying to do something, it will help you do whatever you’re trying to do at an exponentially faster scale.
Uses of ChatGPT
The Managing Director of GreenBook shared an interesting story about his children’s school, a specialty stem kind of Charter School in New Jersey; they’re already drawing the line on this type of technology. They say there is a definite benefit of utilizing such technology, but it cannot be used for tests, writing papers, or real deliverables. It is fascinating that its implications would be different from an educational standpoint. How do we put kind of walls around this? And what are the use cases? Because there are a lot of use cases that could be nefarious and detrimental in many aspects of the world, not just within the research space, I think that’s going to be interesting as well.
While we were promoting the special Live with Dan panel discussion episode on the implications of chatGPT, we sent out a few emails to customers, partners, and the general MRX ecosystem, asking them to tune in. Someone wrote back to me, saying, ” Well, this is really, you know, current because we’re facing something like this in their research studies. Other than patterned responses, one thing that stood out was the response for writing excellent complete sentences that were well thought out and, you know, which doesn’t happen all the time. And you know this isn’t human-written. There is a big challenge – with anything positive, there’s always going to be that negative side too.
The impact of ChatGPT on market research and what QuestionPro is doing about it
While we are speaking about the caveats of ChatGPT and its negative implications, I think there is also a need to talk about how the system impacts market research.
In our world, for every virus, there’s an antivirus. In market research, survey bots, open ends, and other things fall under the gamut of data quality. And a lot is being done to mitigate the impact of lousy survey data on insights management.
At QuestionPro, we’ve used ChatGPT to determine whether you’ve used that ChatGPT in our surveys. For our data quality product, we determine if somebody is using ChatGPT to respond to an open-ended response, so we get a pattern matching around it. If you’ve used ChatGPT to respond to an open-ended comment, we will flag that response, so that’s our short-term solution. Currently, that’s a cat-and-mouse game to some extent, but it’s a big deal to have been able to solve that problem natively.
As with every technology, this could lead to whether we found ChatGPT doing an excellent job grading the outcomes on those open-ended questions. And are we dealing with a lot of false positives?
To provide a little more context about how we are using technology to reduce the impact of insights in the path of Research 3.0, the answer lies in the tool itself. When you add a text comment box, we ask how you can answer the same question differently. Think of five different ways of asking the same question, then look at the answers coming out of ChatGPT, and if there is any pattern matching between somebody’s responses stating that they’re copying and pasting from ChatGPT, we flag it. To further prevent it, let’s say we know that these are the possible list of 2 or 3 ways you could answer this on ChatGPT, so when someone who has the survey changes the question and asks the tool that same question, the tool catches that early on.
There is a threshold on what part of the question or an interpretation of the question is asked to the generative AI platform and what probable responses are. If the threshold crosses a specific limit, 70% or 80%, is copied, then the answer gets flagged. We have already built this into the tool and are working with customers to increase the threshold.
The first part is to build the system to do the catches and start the monitoring. Right now, it’s too early to say how many of these are getting caught since we need a large sample size, but we’ve moved quickly to get this natively ingrained into the system. So in around 30 days, we will share the level of accuracy and what level of importance we are catching right now. In a month or so, we can confidently say, “Well, we’re catching, you know, 80% of the stuff.
However, this is bad or good, depending on which side of the table you’re sitting on to know the impact of ChatGPT on market research.
Now the definite good side is a separate project we are running, which helps people design surveys to get to highly impactful surveys as quickly as possible. Not everyone who uses QuestionPro is a researcher. Due to the nature of the tool, it’s easy even for novices and non-researchers to use it to gather the insights they need. We sell to product managers; we sell to entrepreneurs who have an idea to start a company and want to do some research about this new product they want to build. These people who don’t have large budgets need quick turnaround time responses and agile research and will be significantly impacted by this tech. It is easy to plug into that ChatGPT to get a list of questions about the product that you could ask around usability, flexibility or on, price testing, and more, and that comes back. Then we put that into the survey so we can automatically create a survey in as low as 22 seconds about a particular field. As well as you know, it’ll help them, so you know it’s people having specific use cases on product innovation, pricing, or even due diligence.
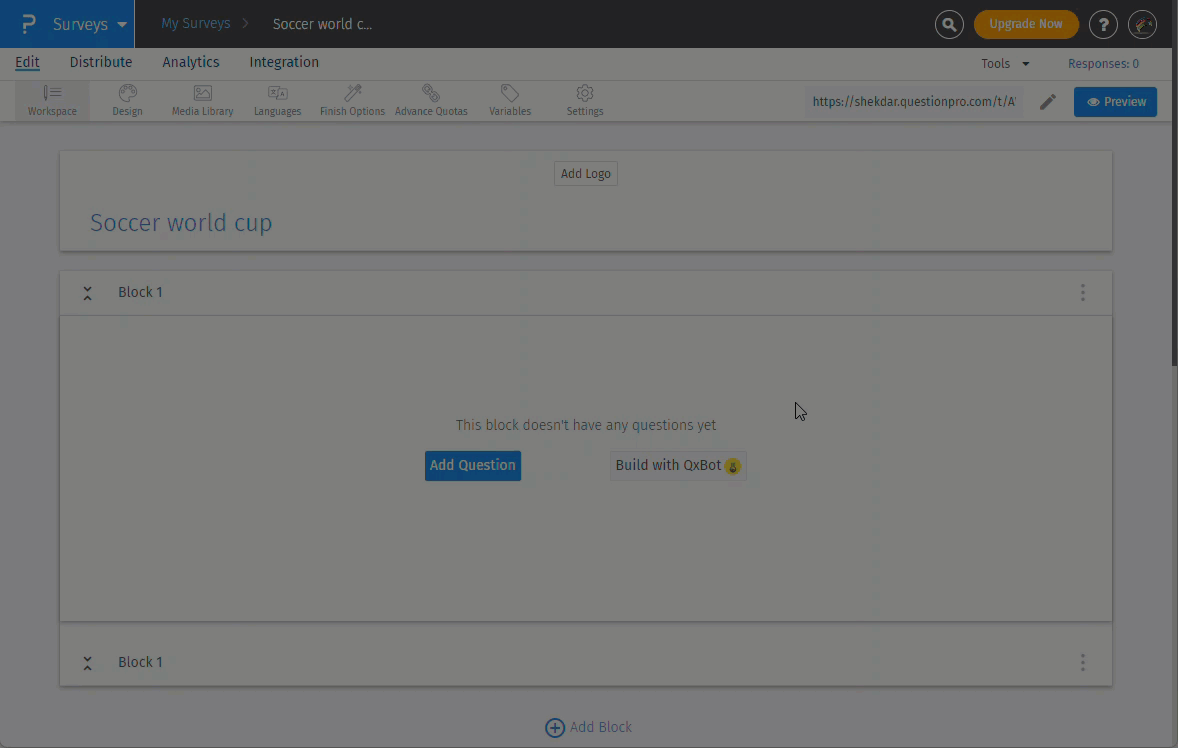
However, the proliferation of technology in market research hasn’t made people creative thinkers. The MRX industry is so process-oriented that researchers are still doing more of the same things, even with an expansion of technology.
What is the impact of digitization and ChatGPT on researchers?
Digitization in research and future research depends on how effectively technology aids market research. Each researcher has a different viewpoint and methodology for approaching insights. With time and the use of technology, expertise expands. With people not leveraging technology, there is a lot of potential disruption in the job market for people not growing their expertise.
ChatGPT may not take you the whole way. Still, suppose it saves considerable time to create a survey or analyze responses. In that case, it can free up time to work on more strategic initiatives, including analysis, posturing, data management, and more.
With technology, you have to be careful that it doesn’t impede you. You have to consider it a way to kill repetitive tasks and improve operational efficacy. But relying solely on it may be counter-productive to your end goal.
This can be best depicted with an example. Content production can be easily automated even before but now so much easier with ChatGPT. It can take on a user persona and make content creation a breeze. However, the flip side of this discussion: can Google recognize that this is ChatGPT reproducing content and, therefore, your SEO strategy won’t work? Nobody knows yet how the algorithm will work. Let’s say you produce a fantastic blog, and Google says oh, this is ChatGPT; therefore, we will downgrade it. At the end of the day, this strategy doesn’t help.
Making technology do the work for you, but smartly, is critical.
Another example of driving home is how researchers can think of ChatGPT in day-to-day tasks. It’s around honing your skill around asking the right question and being able to iterate on those questions. When you ask the right questions and ask for the correct answers, you can get ChatGPT to summarize the key points, surface important information, and even do tasks like rank order from most important to least important, etc. This way, you can have your functional research assistant surface rich insights and create a more compelling story, but the process takes minutes.
Does generative AI impact search-based taxonomy for knowledge management platforms?
Another distinct component of Research 3.0 and the use of technology is knowledge management platforms and insights repositories. With the use of ChatGPT, does it disrupt this model? And should you be worried as a knowledge management platform CEO?
An excellent way to look at this problem statement is that knowledge management (KM) platforms are taxonomies. With ChatGPT, you curate and summarize information reasonably quickly. You can structure the data and put it in the generative AI, and it figures out various ways to slice up data and dress it up any way you like. The implications for every research organization every buyer and organization says that they waste so much previous research that it just goes into a folder and sits there. Companies like Lucy AI have been trying to make a far more efficient process utilizing AI so that you can go through all of your historical data and find railing the points. We’re there now with these technologies allowing you to do just that.
I can ask in the last year what males aged 18 to 24 think about “topic A,” and it will come up with a point of view based on the data reservoir, and that’s pretty remarkable. The infrastructure is such that you don’t know the technology can functionally be bolted on. Now all those projects you’ve got are the asset moving forward instead of having to have them all scripted and constructed. All of a sudden, you don’t have to worry about creating commonality across projects; you can generate discoverability and accessibility, and those are the two things necessary to implement this technology inside of your research. That’s been the Holy Grail that nobody has achieved, and we are on the precipice of being able to accomplish that.
In larger organizations, a lot of time is spent asking the same questions again for different reasons. Now, there is the ability to be agile and iterative across the entire organization and only explore new dimensions of insights that don’t have an answer. Now it also allows the ability to move past just trying to mine past information and start exploring new things that we haven’t gotten to because we’ve just been stuck in data structure and taxonomy.
The knowledge reservoir kind of model where multiple technologies you can query across numerous platforms cross, and so that’s what will be, is a definite win for the researchers as it becomes the common lexicon slash kind of storage. Cross-functional teams can surface insights across languages, studies, methodologies, and more in a commonality.
If you can put all of this tribal knowledge into one AI repository and then query that AI repository, then it’s a huge win. With data tangibility, you can pump data into it with text which is the standard data format. This innovation and a query interface that is more a chat but a human conversational interface make this a game changer.
Knowledge management platforms will be fine until AI repositories across cross-functional tools become a reality. They, however, must evolve to keep up with generative AI and machine learning.
Negative implications of ChatGPT
We’ve spoken in detail about what ChatGPT can do. However, some negative implications are essential to note.
One of the most critical components of this tool is plagiarism. The temptation is always to put a query in the system and paste the response. Algorithms are, in a lot of ways, responsible for how we view the world and how we view data. So those biases and prejudices will naturally find their way into these systems as well. So there is an inherent danger to paste without applying the like research expertise on top to try to eliminate as much of that as possible when expressing a population’s point of view.
To take on an exploratory research standpoint on any given topic, we’re seeing immense fragmentation in online channels. Still, a massive push of expert-led niche content is through platforms like Substack. There’s so much content on topics that needs to be curated and a lot of great stuff out there, but there is also this fragmentation. There’s no central point of truth anymore, and that can create a lot of opportunity and confusion. Depending on the persona that a user takes defines the answer, so in that sense, do generative AI and machine learning have ethics?
Another drawback of ChatGPT is it can really help replace customer service or human beings? A few years ago, chatbots indirectly promised to make customer service reps obsolete. However, recent studies have shown that 97 out of 100 people still want to take to an actual human being. Can you ask your bank a question or if you’re trying to reschedule your flight or ask questions that need emotions? ChatGPT may not have all the answers! Yes, you can request to refine the answer, but the question framing is immaterial if the answer doesn’t exist.
In its nascent stage, another negative implication of ChatGPT in market research is around technical know-how and costs. Karine Pepin, Vice President / 2CV Research has been extensively using GPT-3 to work out different models to work coding open-ended survey responses. Some of the notable things she encountered are mentioned below in her own words:
- To code open-ended responses, since ChatGPT cannot scour data through Google Sheets, using the API model is the only way forward. Even though the API is relatively simple to use, integrating it into tools still requires some technical assembly, and it is not as straightforward as one might expect. And since the GPT-3 trains on the generally available internet knowledge, it cannot understand tribal knowledge related to the survey. As a result, additional survey-context-sensitive information, such as how to code responses or codes to categorize the reactions (i.e. a coding frame), must be included in every API prompt call. Depending on the underlying model used, the cost differs. However, the cost and time factors are very high at a nascent stage.
- It’s possible to create custom versions of GPT models using sample prompts. It is especially useful for classification exercises like coding, as it allows the AI to understand the context of the task through coding examples (i.e. code frame). The quality of the model, however, is still purely subjective.
- ChatGPT can help you identify leading survey questions. However, it is inefficient to input prompts one-by-one since GPT-3 is not scalable. OpenAI’s potential is tied to the underlying GPT-3 engine, which requires some technical knowledge to program and train to improve accuracy in a variety of scenarios.
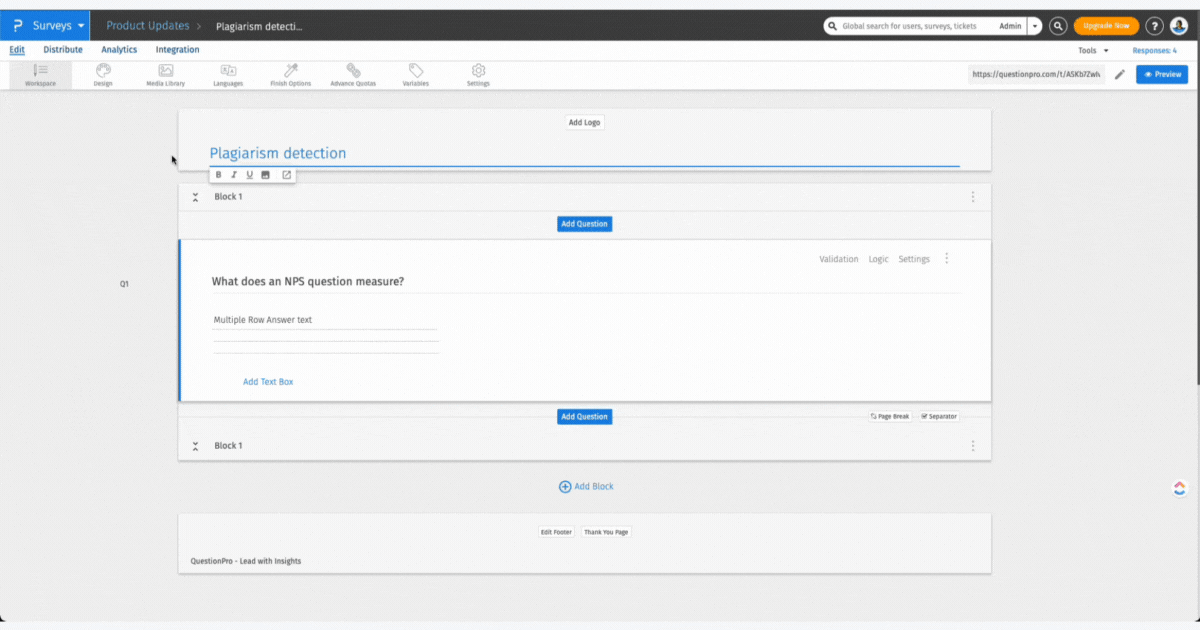
How far back does knowledge need to go to be effective?
In our panel discussion on LiveWithDan, recently, a user was curious that since the knowledge of ChatGPT or GPT-3 doesn’t go beyond 2021, how does that affect the quality of output? And that brings up an interesting point about how market research used to be highly longitudinal in nature but has now evolved post the pandemic to ad-hoc market research. Comparability across data sets has completely changed because the world has changed how we know it! Going from traditional 70% longitudinal research to high-frequency research and discovery, A lot of the research is also DIY to the end that the frameworks are much more in the context of primary market research being gathered today and analyzing that data in the context of that data set as opposed to pulling from historical points of view.
It does bring up an interesting opportunity for innovation if you combine this technology with other predictive technologies. There’s a lot of opportunity to take the historical information and shift it on its head to be more predictive. Brands and researchers want to know what happens next, and if there’s a way to combine past data with that, it’s great.
Regression analysis is a big part of insights management in 2023, where we take stated purchase intent and compare it to similar purchase intent or historical purchase to like products. Then that gives us the weighted, you know, probability of that outcome.
Is ChatGPT a threat to traditional market research or a superpower?
A pertinent question that we have seen murmurs about in the past few days is with ChatGPT, does this make end users and clients self-sufficient and do more themselves or is this a superpower or a mix of both? The short answer is, it definitely is a superpower and it’s path-breaking. Not on the level of the internet but it’s certainly up there. It is definitely an efficiency accelerator and it improves efficiency by an exponential amount.
There are limitless possibilities to leveraging ChatGPT in market research. It is just going to need the community to come together and brainstorm different ideas to make this work for the industry at large.
Does generative AI impact panel and research quality?
There is a case to be made for AI bot panels and the general integrity of traditional panels being threatened by such technologies. Traditional panel supplies do 30% of most research. There is already an issue in dealing with fraudulent responses, bot farms, and other data quality issues in insights management.
Research needs a paradigm shift to move away from purely transactional surveys to more collaborative and conversational forms. Engaging with respondents offers a better experience that’s holistic, iterative and natural and that will go far towards addressing these types of issues.
Conclusion
ChatGPT is fast, easy to use, and highly intuitive in its current form. But it cannot replace researchers and operate in a silo to complete modern tasks like creating a survey to analyze and synthesize insights. There are specific use cases for both qualitative and quantitative market research with a generative AI tool like ChatGPT but the tool is too native, and building models is complicated and not as cost-effective either. This technology definitely puts us well into the path of Research 3.0, but it isn’t perfect yet. Maybe GPT-4 takes us to the next level? All we can currently do is wait and watch.
References
- https://www.linkedin.com/posts/karine-pepin-1802625_chatgpt-mrx-survey-activity-7018939579596476416-W1LX?utm_source=share&utm_medium=member_desktop
- https://www.linkedin.com/posts/karine-pepin-1802625_chatgpt-mrx-activity-7021113906274193408-yDHF?utm_source=share&utm_medium=member_desktop
- https://www.linkedin.com/posts/karine-pepin-1802625_mrx-chatgpt-survey-activity-7021551433720242176-xczS?utm_source=share&utm_medium=member_desktop
- https://www.linkedin.com/posts/adrian-stanica-56a734_market-research-done-in-minutes-when-i-ugcPost-7017350755930046464-9S6I?utm_source=share&utm_medium=member_ios
- https://newmr.org/blog/chatgpt-is-ushering-in-research-3-but-what-is-research-3/
- https://www.greenbook.org/mr/market-research-technology/chatgpt-and-implications-for-market-research/
- http://www.researchnewslive.com.au/2023/01/16/chatgpt-ai-a-market-researchers-best-friend/