Summary
Online surveys are subject to respondent survival bias, which refers to the fact that only the most motivated and engaged respondents are likely to complete the survey, while those who are less interested or have lower levels of engagement are more likely to drop out. This can lead to a sample that is not representative of the population as a whole and may lead to inaccurate conclusions.
If respondents are terminated at a very high rate, it does not make financial sense for the supplier to continue to support sending respondents into your survey.
So, how do you determine what percentage of entrants should be counted as valid and paid for?
Bots are another challenge. As technology advances, bots are becoming more sophisticated and harder to detect.
This article discusses findings from a fraud identification survey, different methods to detect fraud, and how to choose the platform.
Table of Contents
- Introduction
- The sample Goldilocks problem
Introduction
My intention was to do a fair and honest review of data quality, and the blind spots platforms have when it comes to sourcing respondents for surveys. I wanted to find the vulnerabilities in each system and make an argument against why I would or would not do business with a company based on those reasons. I hoped to have a definitive answer for what one should do to circumvent bad actors. What I uncovered was complex and confusing, and I hope you’ll allow me to lay out the data. I’ll consider it a win if you give a second or third thought to owning your own standard of data quality and the cost you may pay for it.
The sample Goldilocks problem
If you ask a buyers of sample, their first honest answer will be, “I do not want a single bad actor in my survey.”
What does that look like for the supplier?
Eventually, zero feasibility.
Suppliers will send respondents to your survey. A decision will need to be made after “in-field” metrics are observed and the following questions (among others) are asked.
- What happened to the first N batch of respondents that I sent in?
- Did they drop out of the survey?
- Did they complete the survey?
- Were they kicked out for questionable behavior?
- Did they terminate?
The answer to these questions determines the future feasibility. If respondents are terminated at a very high rate, it does not make financial sense for the supplier to continue to support sending respondents into your survey. If this is a pattern across several surveys, suppliers will not send their respondents to test the waters of individual surveys, and you will be blocked. Eventually, a large number of suppliers will blacklist you, and you will see few to no entrants. Feasibility will be crushed and costs will skyrocket. Suppliers cannot and will not be predictable for feasibility. The panels will be fighting themselves, burning through resources, churning through valid panelists to fuel the unicorn trap you imagine to be your dream sample.
To a platform like QuestionPro, if we have too many of our customers fielding poorly composed surveys, our relationships with our panelists and external panels will be ruined. Most of our customers will be hurt by a few bad acting accounts. At what point should the platform step in to account for the health of the entire ecosystem? That’s to say, from our point of view, what percentage of bad actors should we allow into my survey not to kill feasibility while delivering valuable, actionable insights I can use to win the hearts and minds of everyone my brand interacts with? The answer is it depends.
Let’s jump into a Goldilocks framework.
If you ask a fictional supplier in their ideal world, what percentage of entrants should be counted as valid and paid for? Their answer to that would be 100%. Understandably that is not realistic when compensation is on the line and doesn’t allow for any error or omission. They’re in the EPCPM/EPCM (Earnings per click per minute) business. This is way too hot.
If you ask buyers to see above, it is way too cold.
If you ask me, my answer is I don’t know. But, I’m willing to help you find the right temperature to thoroughly enjoy your porridge, and as your taste changes, we can adjust that over time as well.
Key findings
- 53.865% red herrings & attentiveness screening questions increase fraud.
- 27.95% of respondents lied about their household income.
- 6.2% of respondents lied about what state they were in, not including neighboring states.
- 4.7% of respondents lied about their age by more than 2 years.
Panel sign-up data vs. Screening vs. In-Survey
Panel sign-up data are the questions initially asked to the participants. These questions are used as targeting criteria.
We can pull signup data from the panel by coding the survey to pass custom variables.
The following questions and answers were checked at three interaction points. This is not a best practice for the respondent experience but necessary as we put together supplier audits.
- We pull from the panel sign-up database, which is updated every two weeks.
- While screening, we asked the same questions and answer choices.
- In the survey too, we asked respondents the same questions and answer choices.
Age, Gender, HHI, State, Relationship Status, Hispanic Yes/No, Employment Status, Industry
We also asked our panels to provide how the respondent was invited to the survey – by either routing or targeting.
Another source of data we looked at was Internet Service Provider residential or mobile, ISP name, host, ASNs, browser, device type, operating system, the brand of the device entering the survey, and model of the device. We checked these values with the ISPs, Panels, QuestionPro technology, 3rd party financial fraud auditing software, and a manual IP check.
Autonomous System Numbers (ASNs)
An IP prefix is a list of IP addresses that can be reached from that ISP’s network. The network operators must have an ASN to control routing within their networks and exchange routing information with other ISPs.
We’ll go into each of the common methodologies to combat fraud in surveys and show you platform-agnostic ways of using your survey tool to achieve the cleanest of responses.
Survey platforms fail to block the most heinous actors from completing your surveys. Here’s what we’ve extensively audited and continue to improve on.
Types of fraud-fighting
Some of the common digital frauds and their ways to counter them are as below.
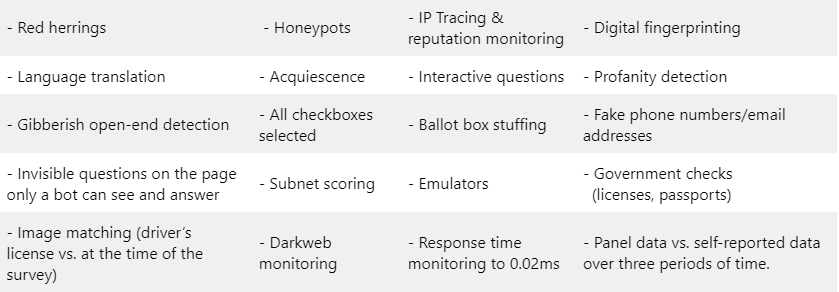
Fraud survey findings
We used survey tools to identify fraud and report on what works to combat that on 10.10.2022.
All data was sourced from a sample aggregator comprising of 20+ panels of at least 20 completed respondents each across the United States.
Control:
Survey 1: No data quality enabled. All QuestionPro data quality checks were turned off.
Fighting 3rd party fraud
- Survey 2: 3rd party APIs using “hands off” fraud fighting to kick out fraud upon survey entry. No misdirection in the survey questions and QuestionPro data quality checks were enabled.
- Survey 3: Misdirections, traditional quality checks such as red herrings, ensuring the survey taker isn’t saying yes to every question, local language 3rd-grade level mastery.
- Survey 4: Red herrings + API detection + Interactive questions (text highlighter, captcha, scales)
All surveys were then audited by finance-level security protocols. The individuals at IP Quality Score have published extensive information on bot detection and honeypots that we will go into below.
Before we proceed further, let’s delve deeper into bot detection.
What is bot detection?
Bot detection is the process of identifying non-human devices and IP addresses that are controlled by automation, such as automated bot attacks or web scraping. This process can be achieved through pattern recognition, device integrity checks, and behavior analysis. Detecting bots is complex as fraudsters use residential proxies and private VPN servers to blend bot traffic with real human traffic.
How to stop unwanted bots?
Prevent unwanted bots by analyzing device behavior and IP address reputation on important pages like sign-up, checkout, or login. Detecting if the device has characteristics of emulators or fake devices are accurate bot detection techniques. Identifying issues like location spoofing, virtual devices, and botnet IP addresses can also be helpful.
How to detect bots?
Detecting bots is easy when looking at the device behavior to analyze if non-human or automated actions are being performed. An intelligent bot manager uses the best device and IP address intelligence data to prevent bots accurately. The IP address can also provide valuable insight by determining if the IP is associated with known bot networks or recent abusive bot behavior.
How to detect bot IP addresses?
Bot IP addresses can be detected by analyzing if abuse has been recently reported or if any non-human bot characteristics of the device are detected. Bot detection is aided by IP address reputation, which looks for suspicious behavior. Bot IPs are usually very abusive and are shared by different groups of bad actors simultaneously.
How to stop bots from scraping websites?
Bots can scrape content and sensitive data from web pages to obtain proprietary data or bypass premium licenses. Prevent bot scraping with a mixture of proxy and VPN detection & device analysis to detect more sophisticated web scraping bots. Patterns of bot scraping include very short page loading periods, limited mouse movement, and excessive page hits within a short period of time. All factors go against how a normal human would browse a website.
Why should you block bots?
Blocking bots is necessary to prevent fake accounts, chargebacks, account takeover, and similar abusive behavior from bad actors. Fraudsters typically automate bot attacks against a website, app, or API. Bot detection can help mitigate fraud and abuse from bad users.
What is a honeypot?
A honeypot is a cybersecurity mechanism that uses a manufactured attack target to lure cybercriminals away from legitimate targets. They also gather intelligence about the identity, methods, and motivations of adversaries.
A honeypot can be modeled after any digital asset, including software applications, servers, or the network itself. It is intentionally and purposefully designed to look like a legitimate target, resembling the model in terms of structure, components, and content. This is meant to convince the adversary that they have accessed the actual system and encourage them to spend time within this controlled environment.
The honeypot serves as a decoy, distracting cybercriminals from actual targets. It can also serve as a reconnaissance tool, using their intrusion attempts to assess the adversary’s techniques, capabilities, and sophistication.
The intelligence gathered from honeypots is useful in helping organizations evolve and enhance their cybersecurity strategy in response to real-world threats and identify potential blind spots in the existing architecture, information, and network security.
What is a honeynet?
A honeynet is a network of honeypots that is designed to look like a real network, complete with multiple systems, databases, servers, routers, and other digital assets. Since the honeynet, or honeypot system, mimics the sprawling nature of a typical network, it tends to engage cybercriminals for a longer period of time.
Given the size of the honeynet, it is also possible to manipulate the environment, luring adversaries deeper into the system to gather more intelligence about their capabilities or their identities.
How does a honeypot work in cybersecurity?
The basic premise of the honeypot is that it should be designed to look like the network target an organization is trying to defend.
A honeypot trap can be manufactured to look like a payment gateway, which is a popular target for hackers because it contains rich amounts of personal information and transaction details, such as encoded credit card numbers or bank account information. A honeypot or honeynet can also resemble a database, which would lure actors that are interested in gathering intellectual property (IP), trade secrets, or other valuable, sensitive information. A honeypot may even appear to contain potentially compromising information or photos as a way to entrap adversaries whose goal is to harm the reputation of an individual or engage in ransomware techniques.
Once inside the network, it is possible to track cybercriminals’ movements to better understand their methods and motivations. This will help the organization adapt existing security protocols to thwart similar attacks on legitimate targets in the future.
To make honeypots more attractive, they often contain deliberate but not necessarily obvious security vulnerabilities. Given the advanced nature of many digital adversaries, it is important for organizations to be strategic about how easily a honeypot can be accessed. An insufficiently secured network is unlikely to trick a sophisticated adversary and may even result in the bad actor providing misinformation or otherwise manipulating the environment to reduce the efficacy of the tool.
Benefits and risks of using a cybersecurity honeypot
Honeypots are an important part of a comprehensive cybersecurity strategy. Their main objective is to expose vulnerabilities in the existing system and draw a hacker away from legitimate targets. Assuming the organization can also gather useful intelligence from attackers inside the decoy, honeypots can also help the organization prioritize and focus its cybersecurity efforts based on the techniques being used or the most commonly targeted assets.
Additional benefits of a honeypot
- Ease of analysis – Honeypot traffic is limited to nefarious actors. As such, the infosec team does not have to separate bad actors from legitimate web traffic. All activity can be considered malicious in the honeypot. This means that the cybersecurity team can spend more time analyzing the behavior of cybercriminals, as opposed to segmenting them from regular users.
- Ongoing evolution – Once deployed, honeypots can deflect a cyberattack and gather information continuously. In this way, it is possible for the cybersecurity team to record what types of attacks are occurring and how they evolve over time. This gives organizations an opportunity to change their security protocols to match the needs of the landscape.
- Internal threat identification – Honeypots can identify both internal and external security threats. While many cybersecurity techniques focus on those risks coming from outside the organization, honeypots can also lure inside actors who are attempting to access the organization’s data, IP, or other sensitive information.
Risks of using a honeypot
- It is important to remember that honeypots are one component of a comprehensive cybersecurity strategy. Deployed in isolation, the honeypot will not adequately protect the organization against a broad range of threats and risks.
- Cybercriminals can also use honeypots, just like organizations. If bad actors recognize that the honeypot is a decoy, they can flood the honeypot with intrusion attempts in an effort to draw attention away from real attacks on the legitimate system.
- Hackers can also deliberately provide misinformation to the honeypot. This allows their identity to remain a mystery while confusing the algorithms and machine-learning models used to analyze activity. It is crucial for an organization to deploy a range of monitoring, detection, and remediation tools, as well as preventative measures to protect the organization.
- Another honeypot risk occurs when the decoy environment is misconfigured. In this case, advanced adversaries may identify a way to move laterally from the decoy to other parts of the network. Using a honeywall to limit the points of entry and exit for all honeypot traffic is an important aspect of the honeypot design. This is another reason why the organization must enable prevention techniques such as firewalls and cloud-based monitoring tools to deflect attacks and identify potential intrusions quickly.
We use a honeypot network that has captured the following demographics data and trends in 24 hours.
- Compare sign-up answers to profiling survey vs pre-screener answer vs answer in the survey question.
- Profiling survey data was pulled in on the link.
- Pre-screener demographic questions were asked by the provider.
- The same questions were asked in the survey.
53.865% Red herrings & attentiveness screening questions increase fraud.
We believe that while making sure your respondents are engaged and ready to answer your surveys, many get unfairly kicked out of the survey due to missed red herrings that aren’t equitable or fair. Respondents were marked inattentive, but when followed up with the actual humans behind the responses, we found that a dog barking or a child crying were typical occurrences mentioned for failing what we believe the easiest of questions.
Humans are fallible, and once kicked out, they are not allowed to give their vote. A fraudulent actor can use an emulator to enter a study 100 times and troubleshoot how to get through the survey. Once one of the bots makes it through the study, they can communicate to the others in the hive and exponentially increase your fraudulent completes. Each bot registers as unique with a different IP address, subnet, and digital footprint.
Why should you rely on experts for fielding? Fighting fraud in market research is a full-time job. Platforms and panel suppliers are, by default, set up to allow more traffic, as that’s how they calculate value. If a platform throttles traffic, it’s a bad experience for all involved. Surveys are slow to get completed causing customers to be late on fielding, which causes them to evaluate the long-term viability of their relationship with the platform.
Why does the platform you choose matter?
Transparency of data quality, longitudinal tracking, and IP & subnet tracking are some of the reasons you should choose a platform carefully.
Most platforms have large volume contracts with fraud circumvention providers, which makes it much more affordable for you, and you do not have to cobble together ten different technologies and know what good looks like. If your platform does not have clear, readily available data quality standards available to you, RUN.
Your platform must allow you to customize data quality thresholds and have a do-it-together mindset behind it. The survey platform should show what they track and highlight the fraudulent actors they are catching. You should be able to tell your survey provider that you’d like to strengthen your tolerance of certain open-ended responses.
No single platform or researcher is perfect, but if you are open to learning from each other, we agree to bring our best selves to the table and be great!