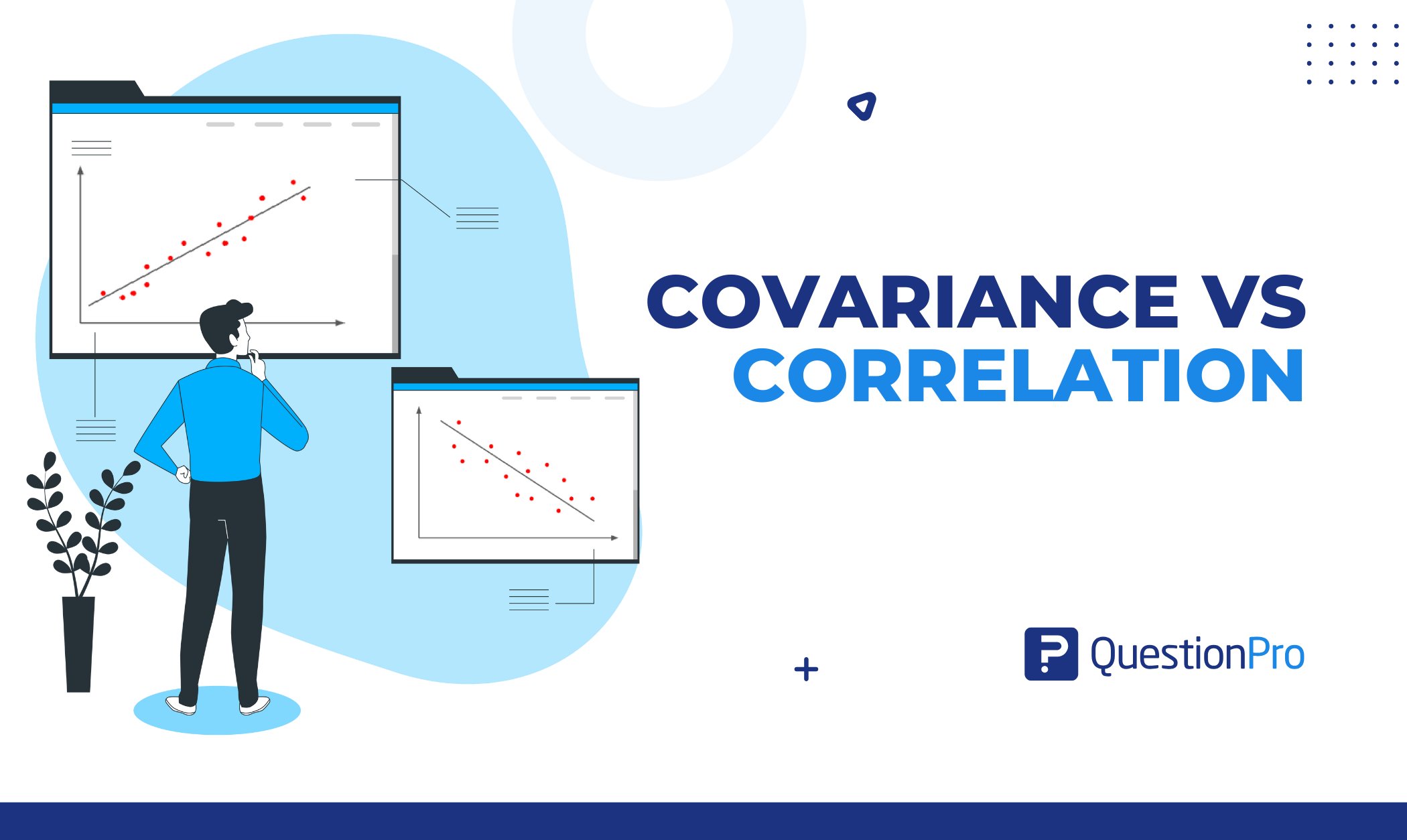
When working with data, we often want to understand the relationship between two variables. Do they move together? Are they connected in some way? Covariance vs correlation helps answer these questions, but they are not the same thing.
In this blog, we’ll break down what covariance and correlation are, how they differ, and when to use each in the simplest way possible.
About Covariance
Covariance is a statistical measure that indicates the extent to which two random variables change together. It reveals whether an increase in one variable would result in an increase or decrease in another variable. Mathematically, the covariance measures between two variables, X and Y, are calculated as the expected value of the product of their deviations from their respective means:
Cov(X,Y) = Σ [ (X − μX) (Y − μY) ]
Where 𝜇𝑋 and 𝜇𝑌 are the means of X and Y, respectively.
Interpretation of Covariance:
- Positive Covariance: If Cov(X, Y) > 0, it suggests that as X increases, Y tends to increase as well, indicating a positive relationship between the variables.
- Negative Covariance: If Cov(X, Y) < 0, it indicates that as X increases, Y tends to decrease, showing a negative relationship.
- Zero Covariance: If Cov(X, Y) = 0, it implies that there is no linear relationship between the variables.
About Correlation
Correlation is a statistical term that describes the relationship between two variables—how one variable changes in relation to another. It indicates whether an increase in one variable would result in an increase or decrease in another variable. The most commonly used measure of correlation is the Pearson correlation coefficient, denoted as ‘r’, which ranges from -1 to 1:
- Positive Correlation (r > 0): As one variable increases, the other also increases.
- Negative Correlation (r < 0): As one variable increases, the other decreases.
- No Correlation (r = 0): No linear relationship exists between the variables.
A correlation coefficient close to +1 or -1 indicates a strong linear relationship, while a coefficient near 0 suggests a weak or no linear relationship. It’s important to note that correlation does not imply causation; a strong correlation between two variables does not necessarily mean that one variable causes the other to change.
Learn More: How a correlation matrix works and how to use it to analyze data.
Key Differences of Covariance vs Correlation
Covariance and correlation are both statistical measures that assess the relationship between two variables, but they differ in several key aspects:
Topic | Covariance | Correlation |
What it measures | Direction of the relationship (positive, negative, or no relationship). | Direction and strength of the relationship (scaled between -1 and 1). |
Scale | Depends on the units of the variables (not standardized). | Always between -1 and 1 (standardized). |
Interpretation | Harder to interpret because values depend on the data’s scale. | Easier to interpret because it’s standardized and comparable across datasets. |
Units | Has units (since it’s based on the original data). | No units (it’s dimensionless). |
Use case | Useful for understanding the direction of a relationship within one dataset. | Useful for comparing relationships across different datasets. |
Example | If covariance is +100, it means the variables move together, but how strongly? | If the correlation is +0.8, it means a strong positive relationship. |
While both covariance and correlation measure the relationship between two variables, correlation offers a standardized, unitless measure that is easier to interpret and compare across different datasets.
Learn More: Different types of Correlation for patterns and relationships.
Covariance vs Correlation: Key Weakness
While both covariance and correlation are useful for understanding relationships between variables, they each have their own weaknesses. Here’s a simple breakdown of their key weaknesses to help you decide when to use (or avoid) them:
Covariance Weaknesses:
- Scale Dependency: Covariance depends on the units of the variables involved. If the units are large or small, the covariance value will reflect that scale. This makes it hard to compare covariances between different datasets with varying units or scales.
- No Standardized Measure: Unlike correlation, covariance doesn’t give you a clear picture of the strength of the relationship. A high covariance value doesn’t necessarily mean a strong relationship because the value is influenced by the scale of the data.
- Difficult Interpretation: The result of covariance can be hard to interpret on its own. For example, a covariance of 100 might seem high, but without knowing the scale of the data, it doesn’t provide much insight into how closely the variables are related.
Correlation Weaknesses
- Assumes a Linear Relationship: Correlation only measures linear relationships between variables. It doesn’t capture more complex, nonlinear relationships (like U-shaped curves). So, even if two variables are strongly related in a non-linear way, correlation might show a weak or no relationship.
- Sensitive to Outliers: Correlation can be heavily affected by outliers. If one data point is far from the rest, it can distort the correlation coefficient, leading to misleading conclusions.
- Doesn’t Imply Causation: Correlation shows whether two variables move together, but it doesn’t tell you if one causes the other. It could be that both are influenced by another variable, and correlation would still show a relationship without any direct cause.
Covariance vs Correlation: Key Strength
Here’s a simple breakdown of the key strengths of covariance and correlation to help you understand when and why to use each:
Covariance Strengths:
- Simple Calculation: Covariance is straightforward to compute. It gives a basic understanding of how two variables change together without needing complicated methods.
- Shows Direction of Relationship: Covariance tells you whether two variables move in the same direction (positive covariance) or in opposite directions (negative covariance). This makes it useful for understanding whether variables are aligned or opposed.
- Useful for Multivariate Data: Covariance is often used in multivariate statistics, such as principal component analysis (PCA) and portfolio theory. It helps identify how multiple variables move together in more complex models.
Correlation Strengths:
- Standardized Measure: Correlation is standardized, meaning it ranges from -1 to +1. This makes it easy to compare the strength and direction of relationships between different datasets, regardless of their scale or units.
- Clear Interpretation: The correlation coefficient gives a clear measure of the strength and direction of a relationship. A correlation of +1 means a perfect positive relationship, -1 means a perfect negative relationship, and 0 means no relationship. This makes it easy to understand the degree of association.
- Widely Used: Correlation is one of the most commonly used statistical tools in fields like finance, economics, and social sciences. It’s useful for a wide range of applications, from determining how variables are related to building predictive models.
Both covariance and correlation have their strengths, with covariance being great for capturing relationships in multivariate settings and correlation offering a standardized, easily interpretable measure of the strength and direction of relationships.
Learn More: Correlational Research with Examples.
Covariance vs Correlation: Which is better?
When deciding between covariance and correlation, it’s important to understand that both have their advantages, but which one is “better” really depends on what you’re trying to achieve.
When to Use Covariance?
Covariance is best used when:
- You need to understand how two variables change together, especially in more complex analyses like portfolio theory or multivariate statistics.
- You’re not concerned with the scale or units of the data. Covariance works well when you just want to see if two variables move in the same or opposite direction without worrying about the exact strength of their relationship.
But, covariance has its limitations:
- It can be hard to interpret because the result depends on the scale of the data (e.g., if the units change, so does the covariance).
- It doesn’t tell you how strong the relationship is in a clear, standardized way.
When to Use Correlation?
Correlation, on the other hand, is generally preferred when:
- You want to measure the strength and direction of a relationship in a standardized way, where you don’t have to worry about the units of the data.
- You need a result that’s easy to interpret. A correlation value close to +1 or -1 tells you that the relationship is strong and positive or negative, and a value near 0 shows no relationship.
- You’re working with datasets that may vary in units or scale.
But, correlation also has its own downsides:
- It assumes that the relationship between the variables is linear, so it won’t work well if the relationship is more complex (e.g., curved).
- It can be sensitive to outliers, which might distort the results.
Which is Better?
It depends on the context:
- If you’re working with data in the same units and just want to know if two variables move together, covariance can be useful.
- If you need a clear, easy-to-interpret measure of how strongly two variables are related, and you want that relationship to be scale-independent, then correlation is probably your best choice.
In most practical scenarios, correlation tends to be more commonly used and preferred because it’s standardized and easier to interpret. However, covariance is still important, especially in more advanced analyses where relationships among multiple variables are involved.
Learn More: Identify Linear Relationships Between Variables Using Our Correlation Analysis Tool!
Try QuestionPro For Correlation Analysis
If you’re looking to dive into correlation analysis and need a tool that makes it easy, QuestionPro could be the perfect fit for you! Here’s why QuestionPro is a great choice when you’re focusing on correlation analysis:
- Built-In Tools: QuestionPro has a Correlation Matrix tool that automatically calculates correlation coefficients for your survey data. No need for manual calculations or complex formulas.
- Visualizations: The tool provides clear visualizations like heatmaps, making it easy to spot strong or weak relationships in your data at a glance.
- Handles Survey Data Perfectly: If you’re working with survey data (e.g., ratings, scales, or multiple-choice responses), QuestionPro is designed to handle it seamlessly. It can analyze relationships between variables like satisfaction scores, demographics, or behavioral data.
- Saves Time: Instead of exporting data to Excel or other software, you can do everything within QuestionPro. This saves time and reduces the risk of errors.
- Actionable Insights: QuestionPro doesn’t just give you numbers—it helps you interpret the results. For example, if you find a strong positive correlation between customer support quality and loyalty, you can focus on improving support to boost loyalty.
Conclusion
Both covariance and correlation help us understand relationships between variables, but they serve different purposes. If you’re analyzing data, knowing when to use covariance vs correlation can make your insights much more meaningful.
Would you like an easier way to analyze data? Try QuestionPro, a powerful platform that helps you collect, analyze, and interpret data effortlessly!
Frequently Asked Questions (FAQs)
Answer: No, covariance is not the same as correlation. Covariance indicates the direction of the relationship between variables, but correlation standardizes this value, making it more useful for comparing the strength and direction of relationships across different data sets.
Answer: Covariance and correlation both measure the relationship between two variables, but covariance provides a measure of direction (positive or negative), while correlation standardizes the relationship to a scale of -1 to 1, making it easier to compare across different datasets.
Answer: No, covariance doesn’t provide a standardized measure of strength, unlike correlation. Correlation, with its scale from -1 to 1, is a better indicator of the strength and direction of a relationship.
Answer: Correlation is essentially a normalized version of covariance, where the covariance value is divided by the product of the standard deviations of the two variables, making it easier to interpret the strength of their relationship.
Answer: Yes, both covariance and correlation can be zero. Zero covariance indicates no directional relationship, and zero correlation means there is no linear relationship between the variables.