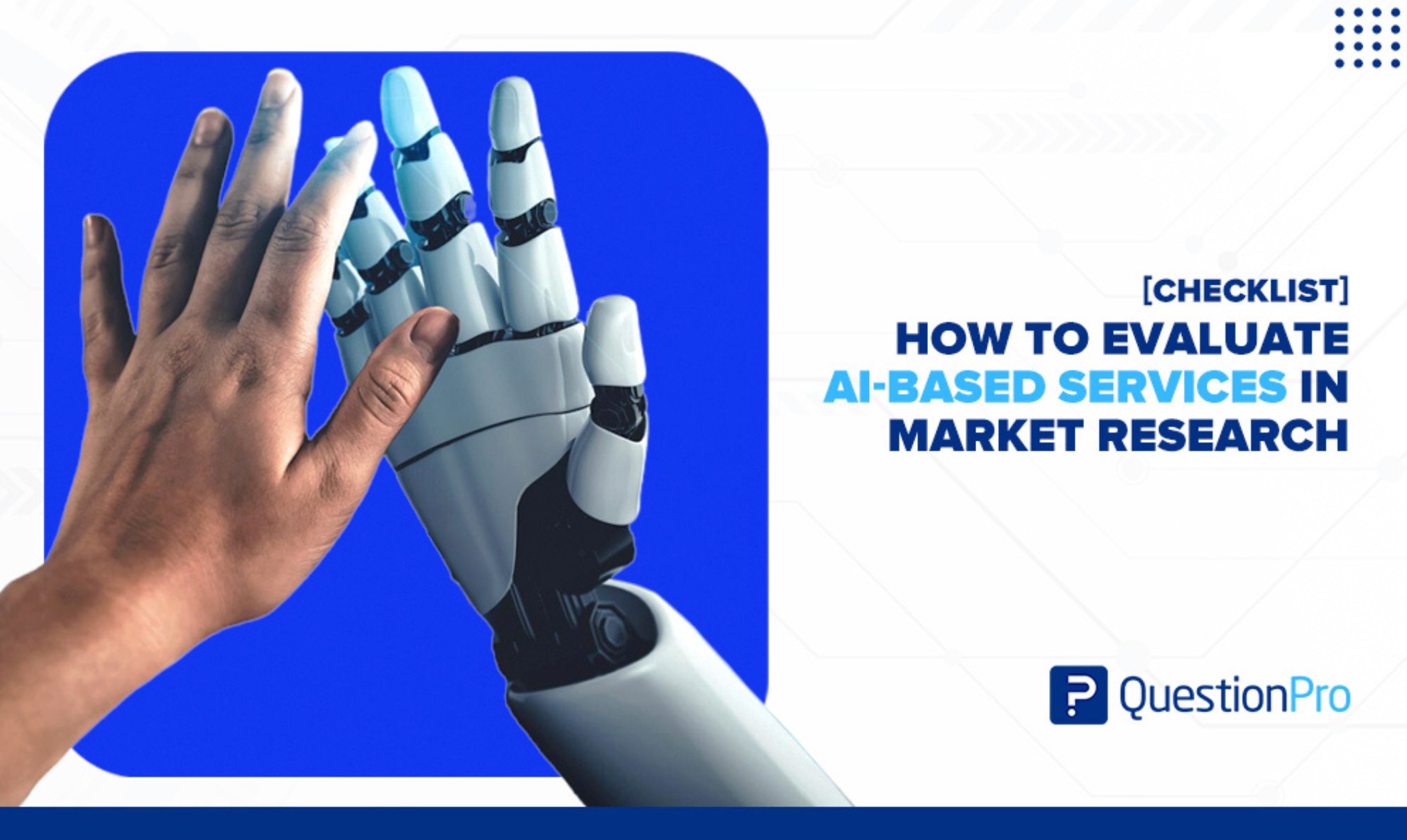
In March 2024, ESOMAR launched a document to help the industry make more informed decisions when procuring AI-based solutions.
This is an exciting development as it will help insights professionals identify suppliers that meet the standards needed for a trusted and robust service. ESOMAR has laid down 20 questions organizations should seek answers to from providers of AI tools.
The goal of this write-up is to identify the organizational behaviors that contribute to their ability to meet the expectations laid down by ESOMAR. I have also summarized the questions into 3 easily understandable and logical sections. Finally, I have compiled a simple checklist to make it easier for organizations to conduct an assessment.
I am a big believer in organizational values. What organizations share about their beliefs, how they posture externally, and what they push internally says a lot about them.
At an overall level, here are the values AI suppliers need to exhibit in all their interactions with you. In my view, this will support the assessment you are going to make based on ESOMAR guidelines:
- Openness – How open are they to answering your questions, showing how they have done what they have done, where they use third-party data, and most importantly, where they believe they are lacking and need to do better? Building the required safeguards around the proper application of AI is complex, cumbersome, and expensive. You need to trust that your partner is doing the right things and is on the right track.
- Simplicity – Watch out for excessive use of complex terminologies or convoluted explanations and answers to simple questions. These are usually signs that the AI supplier does not understand the offering and has relied heavily on third-party or open AI. Another issue could be that they do not have the necessary safeguards in place.
- Continuous Improvement—You need to see evidence that the AI supplier has an internal continuous development and improvement process. The necessary investment and effort to independently review internal protocols is part of the organizational culture.
ESOMAR guidelines are split into 5 sections:
- A. Company profile – gain an initial understanding of the credentials of the supplier organization.
- B. Is the AI capability/service explainable and fit for purpose? Whether the capability aligns with their business purpose and is likely to provide a clear benefit.
- C. Is the AI capability/service trustworthy, ethical, and transparent? Whether the buyer and supplier are aligned on ethical principles, potential biases, data security, and resilience.
- D. How do you provide Human Oversight of your AI system? Understand how human involvement and oversight have been considered in both the development and the operation of the AI applications on offer.
- E. What are the Data Governance protocols? Can be used in combination with the other questions in this guidance or as a standalone checklist.
After an in-depth reading of the ESOMAR report, we look at 3 key themes. What ESOMAR is really asking organizations to do is to answer 3 questions, all of which are pivoted in the notion of ‘Trust’:
- Do you trust the company supplying AI solutions?
- Do you trust the process the company uses to build its AI products?
- Do you trust that your data will be protected?
Not all buyers of AI services may be well-versed with all the nuances related to this topic. Much of their assessments would eventually be dependent on the declarations made by the supplier. Therefore, there is a need to identify ways in which anyone, with or without technical knowledge, can perform a sound assessment.
At the end of the document, you will find a simple-to-use checklist organized to help you answer the three questions laid out above.
- How to trust the company supplying AI solutions?
Before evaluating the product, ESOMAR suggests looking at the people who have built the AI product. I believe this is the most important aspect of all the others they identified.
A lot of times, companies seeking AI solutions don’t necessarily fully understand what it is that they are buying, and they end up in two likely scenarios when selecting a supplier:
- What vs. Who— decisions get made based on “what” is said and not so much on ‘who’ is saying it. This basically means that telling a good story or showcasing something that ‘looks’ good gets equated to being the ‘right’ solution.
- Who vs. What—Sometimes, companies delegate their burden of evaluation by choosing a well-named or large global organization. They don’t consider the possibility that a smaller, less well-known company may have done a better job and provided the same solution but at a better economic value.
ESOMAR encourages you not to make these common mistakes and has identified criteria or questions for evaluating a supplier.
2. How to trust the AI product offered?
We have been living with AI long enough now that the novelty has worn off. There is a higher degree of recognition of risks and limitations of using AI solutions.
However, research companies still lack clarity about how to evaluate an AI offering effectively, what they should look for, and how to avoid making the most common mistakes.
In this regard, the key points highlighted by ESOMAR present a robust path for such an evaluation. The guideline covers aspects like:
- Explainability
- Use of 3rd party apps
- Use of data
- Ethics/Transparency
- Duty of care
- Human oversight
- GenAI specific issues
- How can we trust that data will be protected?
There is no conversation about ethical AI without discussing the use of data. While the importance of data and ways to assess guardrails around its use have been mentioned in earlier sections, this section goes into several technical details.
There are specific guidelines around how to assess data quality, lineage, sovereignty, ownership and compliance.
If you want to know more, we have a blog about Top 5 AI Tools for Business and Uses for Research
Conclusion
AI is revolutionizing the market research field. There have been many successful applications of GenAI to optimize key research processes and derive uncommon insights from data faster and better than in the past.
However, these applications come with a degree of risk. As responsible market researchers, we must ensure that we demonstrate a reasonable duty of care when selecting AI suppliers.
Checklist for buyers of AI services
To facilitate buyers of AI services in market research, I have developed an easy checklist on the basis of ESOMAR’s 20 questions.
I hope that this document will make the assessment process easier. I have combined the checklist across the 3 sections I had identified earlier in this document.
- How to trust the company supplying AI solutions?
Area of assessment | Checklist | Answer (Yes / No) |
Know-how and experience in AI for MR | Have they successfully implemented AI solutions for other clients? | |
Do they have a healthy conversion rate? | ||
Are users of their AI services primarily market researchers and insights professionals? | ||
Have they had academic reviews of their model development processes? | ||
Have they mapped the end-to-end market research cycle to identify opportunity areas for AI? | ||
Vision and understanding of pain points | Do they have a documented AI vision? | |
Have they mapped the end to end market research cycle to identify opportunity areas for AI? | ||
Have they clearly identified the problems they want to solve with AI? | ||
Have they set optimization targets? | ||
Do their plans include an understanding of emerging trends in market research? | ||
Openness | Will they share profiles of their team involved in building AI products? | |
Do they share what has not worked for them? | ||
Have they shared major security breaches or other critical incidents that happened in the past 3 months? |
- How to trust the AI product offered?
Area of assessment | Checklist | Answer (Yes / No) |
Explainability | Do they proactively mention the names of tools, models, and directories they have used? | |
Can a non technical person in your team understand how they have developed the AI solution? | ||
Based on your assessment of how much of their performance is dependent on such third-party apps, are you confident about the continuous availability of key functionalities? | ||
Use of 3rd party apps | Do they tell you that they have used 3rd party apps? | |
Do they have enterprise deals with such 3rd parties that cover areas like data security, use of data, and identification of risks (especially privacy-related)? | ||
Have you validated the model output with original data and used your own common sense logic to detect hallucination (GenAI creating data/facts that do not exist)? | ||
If they are using 3rd party apps and models, have they talked about building native models? | ||
Use of data | Do they anonymize data used for modeling? | |
When asked do they share what has not worked? | ||
Have they shared major security breaches or other critical incidents? | ||
Ethics / Transparency | Do you believe you have the option to opt in/out in regards to using your data for training their models? (This is not relevant when they are training models specifically for your use, for e.g. your industry or reporting according to your Taxonomy) | |
Have they demonstrated how they mask any PII data, if applicable? | ||
Do you believe they are open to communicating potential risks and exposures? | ||
Do they comply when asked to share necessary information and documentation? | ||
Duty of Care | Have they shared their privacy policy with you? | |
Do they have an AI governance document? | ||
Is this information available to all their employees as well as shared via their website? | ||
Human oversight | Do you get a sense that they mainly used unsupervised learning? | |
Do they have processes for human review and validation during the model development process? | ||
Have they invited you to be part of the iterative model development process such that you get to review the model outputs? | ||
Do they have independent reviews of model outcomes? | ||
Do they use humans for data labeling? | ||
GenAI specific issues | Have they informed you about the use of synthetic data during model training? | |
Have you validated the model output with original data and using your own common sense logic to detect hallucination (GenAI creating data/facts that do not exist)? | ||
Do you feel that the supplier takes GenAI-related issues seriously? | ||
When asked, do they share what has not worked? |
- How can we trust that data will be protected?
Area of assessment | Checklist | Answer (Yes / No) |
Data Quality | Have you detected any data used in model training that you consider to be biased or incomplete (for e.g. data from fake or irrelevant review sites)? | |
Do you have the ability to prevent the supplier from using all or part of your data for model training purposes? | ||
Do you believe the supplier has used synthetic data with no restrictions or review? | ||
Data Lineage | Are you confident that they have put sufficient controls in place to ensure that they have the right to use the data? | |
Has the supplier even been penalized for a breach of local data protection laws? | ||
Compliance with data protection laws | Are you confident that they have put sufficient controls in place to ensure they have the right to use the data? | |
Do you have the ability to request the supplier not to use certain data for their model training? | ||
Have you been asked to give your consent for the use of your data? | ||
Data Ownership | Is it always evident to you that you have full ownership of your data and how it’s used? | |
Do you have the ability to request that the supplier not expose certain data to openAI (e.g., ChatGPT)? | ||
Does the supplier provide sufficient flexibility about where to host your data (check if they have local servers in your preferred markets)? | ||
Data Sovereignty | Do you have the ability to prevent the supplier from using all or part of your data for model training purposes? | |
Do you have the ability to not allow all or a part of your data to be used by the supplier for model training purposes? |
Are you interested in learning more about the impact of AI-based services in Market Research and their influence on the industry? We invite you to revisit our latest webinar, in which Dan Fleetwood and Sumair Sayani discuss the pros and cons of this emerging technology.