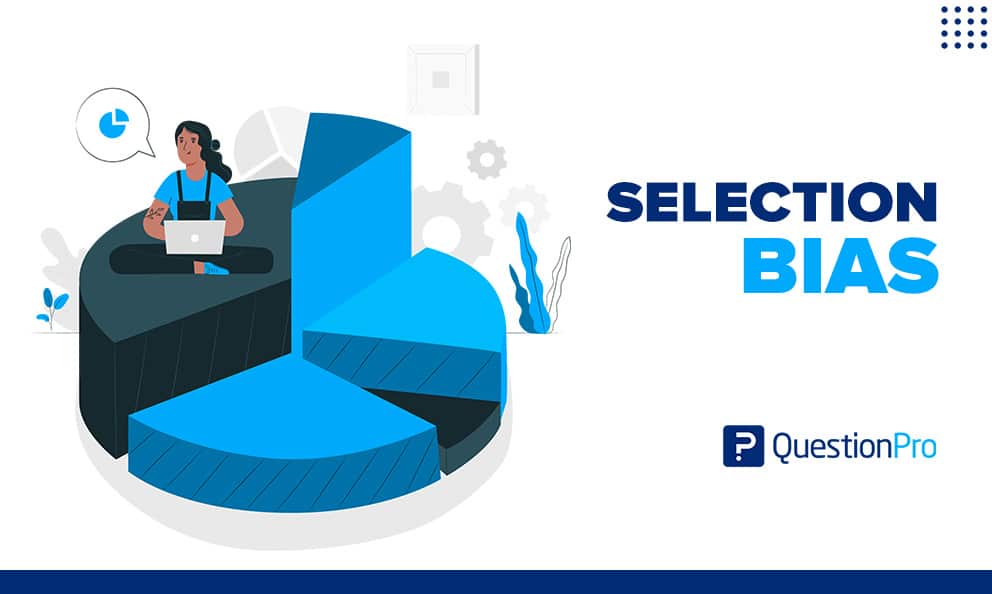
Researchers may need help with findings that don’t match the realities of the target community. There are numerous causes, but selection bias is the most important. It occurs when the study sample needs to accurately represent the population of interest, resulting in variations in the research results.
Understanding selection bias, its practical impacts, and the best ways to avoid it will help you deal with its effects. Everything you need to know about how to enhance your data collection process will be covered in this post.
What is Selection Bias?
Selection bias refers to experimental mistakes that lead to an inaccurate representation of your research sample. It arises when the participant pool or data does not represent the target group.
A significant cause of selection bias is when the researcher fails to consider subgroup characteristics. It causes fundamental disparities between the sample data variables and the research population.
Selection bias arises in research for several reasons. If the researcher chooses the sample population using the incorrect criteria, they may find numerous examples of this bias. It may also happen due to elements affecting study volunteers’ willingness to participate.
All statistical models in the learning sciences require data. Good data is crucial to developing a statistically valid set of models, but it’s surprisingly easy to get insufficient information. Selection bias affects researchers at all process stages, from data collection to analysis.
For instance, researchers may need to realize that their findings may not apply to other people or different settings. This type of error presents individuals randomly assigned to one of two or more groups, yet, only some people who can be enrolled actually participate.
This means that people considered suitable candidates for a particular program may or may not choose to participate. Thus, those who do participate in the program may have different characteristics than those who do not. The existence of the non-random selection process can lead to incorrect inferences about causation and statistics related thereto, as well as invalidation of gathered data.
We have published a blog that talks about subgroup analysis; why don’t you check it out for more ideas?
Selection Bias Types
There are many types of selection bias, each and every one of them impacting the validity of your data in a specific way. Let’s go over some of the most common ones:
- Sampling Bias:
Sampling bias is a form of selection bias that occurs when we don’t collect data from all the people who could be in our population on a crucial variable. Some of the reasons for this could be that the researcher gathers their sample mostly from convenience or convenience sampling, or sometimes by carefully selecting individuals who are similar and have similar characteristics to study subjects but have yet to be randomly chosen from their population.
This can skew any statistical analysis and understanding of the results in that particular case
Read more: Bias in Research by QuestionPro
- Self-selection Bias:
This type of selection bias, also known as “Volunteer bias,” occurs when people who choose to participate in a study are not representative of the larger population of interest. For example, if you want to study student preferences for careers, you may only be able to attract students from schools known for attracting wealthy students. Volunteer bias may also occur when a study examines people of a certain race but doesn’t have enough participants who identify as members of that race.
Like any other form of bias, self-selection bias distorts the data gathered in research. In most cases, the researcher will end up with highly inaccurate results and the non-existing validity of systematic research.
- Nonresponse bias
Nonresponse bias happens when people don’t answer a survey or participate in a research project. It often happens in survey research when participants lack the appropriate abilities, lack time, or feel guilt or shame about the topic.
For Example, Researchers are interested in how computer scientists view a new piece of software. They conducted a survey and found many computer scientists didn’t respond or finish.
Researchers found that the respondents believed the software was excellent and high-quality after receiving the data. However, they discovered that they received mainly unfavorable criticism after releasing the new software to the full population of computer scientists.
The survey participants were entry-level computer scientists who couldn’t spot program flaws. The survey respondents did not reflect the more significant computer scientist population. Hence the results were inaccurate.
- Exclusion Bias:
Inclusion bias happens when the researcher intentionally includes some subgroups in the sample population. It is closely related to non-response sampling bias and affects the internal validity of your systematic investigation.
Experts define inclusion bias as “the collective term covering the various potential biases that can result from the post-randomization inclusion of patients in a trial and subsequent analyses.” When this happens, your research outcomes may establish a false connection between variables.
Exclusion bias occurs when you intentionally exclude some subgroups from the sample population before randomizing them into groups. You may have excluded patients with certain conditions, such as cancer or HIV/AIDS, because it would have been unethical to study those people without their consent. Or, maybe you excluded them because you didn’t want to give them access to another treatment option during their clinical trial. Some researchers also choose not to include people who are too ill or too old for participation in clinical trials (because these people might not be able to participate effectively or might not receive enough benefit from participating).
- Recall Bias:
One of the most common forms of recall bias is retroactive memory distortion. Retroactive memory distortion occurs when people remember events and experiences in a way that suits their current needs rather than their original purpose. For example, someone might recall an event as having been a positive experience or even enjoyable if it was meant to be negative. In addition, retroactive memory distortion can occur when people have difficulty remembering details that are important to the research topic, such as facts about their own lives or the lives of others.
Retroactive memory distortion can also occur when people include inaccurate information in their recall reports. This happens when they report something that never happened or something that happened at a different time than when it actually occurred.
For example, a person might report that he spent five hours traveling from work to home on a particular day when in reality, it only took him three hours because he had lunch at his desk beforehand and forgot about it until later in the day.
- Survivorship bias
Survivorship bias occurs when a researcher subjects variables to a screening contest and selects those who successfully complete the procedure. This preliminary selection method eliminates failed variables because of their lack of visibility.
Survivorship bias focuses on the most successful factors, even if they don’t have relevant data. It can alter your research outcomes and lead to unnecessarily positive views that don’t reflect reality.
Suppose you’re researching entrepreneur success variables. Most famous entrepreneurs didn’t finish college. It could make you assume that leaving college with a strong concept is enough to launch a career. But the majority of college dropouts don’t end up rich.
Actuality, many more people dropped out of college to launch unsuccessful businesses. In this example, survivorship bias occurs when you only pay attention to dropouts who succeeded and ignore the vast majority of dropouts who failed.
- Attrition bias
Attrition bias occurs when some survey respondents drop out while it is still being conducted. As a result, there are many unknowns in your research findings, which lowers the quality of the conclusions.
Most of the time, the researcher looks for trends among the drop-out variables. If you can identify these tendencies, you might be able to determine why the respondents left your survey suddenly and take appropriate action.
- Undercoverage bias
Undercoverage bias arises when a representative sample is drawn from a smaller proportion of the target population. Online surveys are especially vulnerable to undercoverage bias.
In an online survey on self-reported health, let’s say you focus on excessive drinking and smoking behaviors. Although, because of your way of conducting the survey, you are deliberately excluding people who don’t use the internet.
This way, older and less educated individuals are left out of your sample. Since internet users and non-users differ significantly, you can’t draw reliable results from your online survey.
How to Avoid Selection Bias
Estimating the strength of a relationship between an outcome (the dependent variable) and several predictor variables is essential to many research questions. Bivariate analysis and multi-regression analysis methods are commonly used to avoid selection bias.
Bivariate analysis is a quantitative analysis often used to determine the empirical relationship between two variables. In this method, researchers measure each predictor variable individually and then apply statistical tests to determine whether it affects the outcome variable.
If there is no relationship between the predictor variables and the outcome, then they will not be able to find any evidence of selection bias in their data collection process. However, if there is some sort of relationship between these variables, then it may be possible that there was some level of selection bias present when collecting this data.
Multi-regression methods allow researchers to assess the strength of this relationship between an outcome (the dependent variable) and several predictor variables.
There’s a good chance you affected your survey results through selection bias. Review the following advice to help you avoid selection bias:
During survey design
Try some of these suggestions to avoid selection bias when you are developing the structure for your survey:
- Make sure that your survey objectives are apparent.
- Specify the standards that should be met for your intended audience.
- Allow every possible participant a fair opportunity to take part in the survey.
During sampling
Consider putting some of these strategies into practice during the process of selecting samples:
- When employing random sampling in your processes, ensure proper randomization.
- Be sure that your list of participants is up to date and accurately represents the intended audience.
- Make sure that the subgroups represent the population as a whole and share the essential factors.
During evaluation
When going through the evaluation and validation process, you need to think about putting some of these ideas into action to avoid selection bias:
- If you want to ensure that your sample selection, procedure, and data collection are free of bias, having a second researcher look over your back is a good idea.
- Apply technology to monitor how the data changes so you may identify unexpected outcomes and investigate quickly to repair or avoid inaccurate data.
- Check previous fundamental research data trends to verify if your research is on track for strong internal validity.
- Invite the people who didn’t answer the survey to an additional one. A second round might yield more votes for a clearer understanding of the findings.
Learn how to avoid selection bias with this quick Audience by QuestionPro video!
What are the impacts of selection bias?
There is always the possibility of random or systematic errors in research that compromise the reliability of research outcomes. Selection bias can have various impacts, and it’s often hard to tell how significant or in which direction those effects are. The impacts can lead to several issues for businesses, including the following:
- Risk of losing revenue and reputation
For business planning and strategy, insights obtained from non-representative samples are significantly less helpful because they don’t align with the target population. There is a risk of losing money and reputation if business decisions are based on these findings.
- Impacts the external validity of the analysis
Research becomes less trustworthy as a result of inaccurate data. Therefore, the analysis’s external validity compromises because of the biased sample.
- This leads to inappropriate business decisions
If the final results are biased and unrepresentative of the topic, it is unsafe to rely on the study’s findings when making important business decisions.
Conclusion
Understanding selection bias, its types, and how it affects research outcomes is the beginning step in working with it. We’ve discovered crucial data that will help in identifying it and working to reduce its impacts to a minimum. You can avoid selection bias by using QuestionPro to gather reliable research data.
Various situations can result in selection bias, such as when non-neutral samples are combined with system problems. An enterprise-grade research tool to use in research and alter experiences is the QuestionPro research suite.
QuestionPro Audience can help you collect valuable data from your ideal sample.
When conducting research, it’s essential to understand the nature of selection bias. This is the tendency for your research results to be influenced by the characteristics of your participants or sample.
If you’re conducting a study on the effects of sugar on diabetes, for example, and you have a group of people with diabetes who are all members of your church, that could be a source of selection bias. They may be more likely to participate in church activities than those who don’t have diabetes, therefore, more likely to find themselves in the sample.
If you want to avoid this kind of bias in your study, you should collect data from a wide variety of reliable sources with QuestionPro Audience