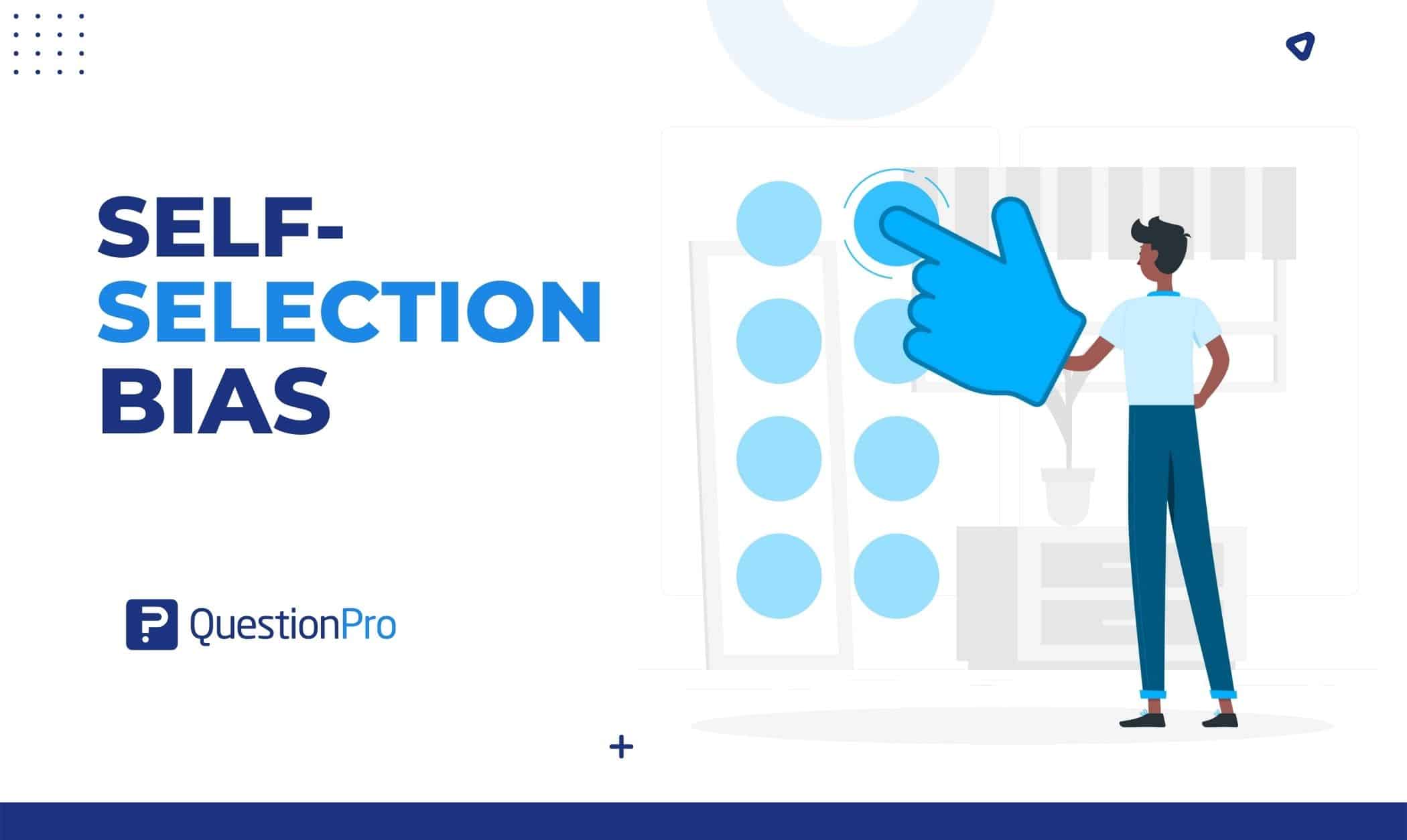
Most of the time, researchers have trouble getting results that don’t match up with what happens in the target population. Even though there are many reasons for this, self-selection bias is one of the most important ones.
When we say “selection bias,” we mean that an experiment went wrong that caused your population of interest to be misrepresented or underrepresented.
This bias is a problem when researching programs or products. Self-selection makes it hard to do market research and evaluate programs.
In this blog, we will go through the definition of self-selection bias and what methods we should follow to reduce bias, and we will also give some examples of this bias.
What is self-selection bias?
Self-selection bias happens when people choose to join a group on their own. It causes a biased sample when nonprobability sampling is used. It is often used to describe situations in which the traits of the people in the group, which led them to choose to be there, lead to strange or bad things happening in the group.
It is similar to the non-response bias, which is when the group of people who answered the survey gave different answers than the group who didn’t answer.
Now we will discuss the methods of reducing this bias. We will also give some examples of it as well. To learn more, stay with us till the end.
Methods of reducing self-selection bias
The most obvious way to eliminate self-selection bias is not to let people choose themselves for a survey. To get a sample, a probability sampling bias technique is ideal.
Probability sampling method
Probability sampling is a method for choosing a population for a systematic study based on probability theory. Here, the researcher picks a small group of people from the whole population whose characteristics they want to estimate.
Probability sampling is based on the randomization principle, which means that all members of the research population have an equal chance of being in the sample population.
For example, if the population size is 500, every person in the population has a 1 in 500 chance of being in the research sample.
The basic idea behind this method is that if you can pick a random sample representative of the whole, your estimates will be accurate. When the sample population is big enough, you can use statistical techniques to conclude the whole population based on the sample.
Here are some examples of probability sampling methods:
- Simple random sample: The American Community Survey collects information about life in the United States by randomly picking people.
The United States Census Bureau asks a random sample of people in the country for detailed information. They then use this information to conclude the whole population.
- Systematic sampling: Systematic sampling is a type of probability sampling in which the researcher uses a random starting point and fixed intervals to find members of the research population. Here’s what’s happening right now. Example of systematic sampling
Let’s say the people you’re interested in number 800. You can pick the sixth person randomly as your starting point and choose a random sampling interval of 10 people. It means that the research population will be made up of every 10th element in a row.
- Stratified sampling: The idea of stratification is what makes stratified random sampling work. When the study population is split into subgroups (called “strata”) based on gender, age, income level, and other similar factors, this is called “stratification.” Each layer is given a weight based on how big it is. Then, a sample is picked by giving each stratum a random place to start.
- Cluster sampling: Cluster sampling is a way to choose research samples from a large population based on chance. In this case, the researcher divides the population into existing groups, such as neighborhoods and cities. It is also referred to as multi-stage sampling.is also known as multi-stage sampling.
To cluster a research sample, the researcher divides the sample into naturally occurring subgroups with different traits. Next, they pick clusters at random to use as samples and get the needed information.
LEARN ABOUT: Selection Bias
Examples of self-selection bias
The following examples show a few situations where self-selection bias is likely to happen:
Example 1
A teacher wants to know if a new course on how to do well on tests helps students do better. She puts a sign-up sheet outside her classroom and lets students decide for themselves if they want to take the class.
Self-selection bias is likely because students who are more serious about school are more likely to sign up. It means that the sample of students who take the course probably doesn’t look like the whole group who could take it.
Example 2
Imagine that a local government sends out a survey asking people if street signs should also be written in languages other than English to make it easier for people who don’t speak English to get around.
Self-selection bias is likely because only residents who can read English will answer the survey. It means that the opinions of the people who answered the survey are probably not the same as those of all the people who live in the town.
Example 3
If a biologist wants to figure out how tall a specific species of deer are on average, she might put deer food in an open meadow and take pictures of the deer that come to eat it.
In this case, self-selection bias is likely to happen because only the deer who like that type of deer food or are more comfortable being out in the open is likely to enter the meadow and be included in the sample data.
So, it’s unlikely that the average height of the deer in this sample will be the same as the average height of all deer.
LEARN ABOUT: Theoretical Research
Conclusion
We learned about self-selection bias and methods of how to reduce this research bias. Also, we gave some examples of it. Self-selection bias is a big problem in research. It makes a biased sample when nonprobability sampling is used. We discussed the probability sampling method, which can help prevent this bias in your business.
QuestionPro is much more than just survey software; we offer a solution for every problem and business. We also have data-management platforms, such as our InsightsHub research library.
Organizations worldwide use knowledge management systems and solutions like InsightsHub to manage data better, save time to acquire insights, and enhance historical data usage while lowering costs and increasing ROI.