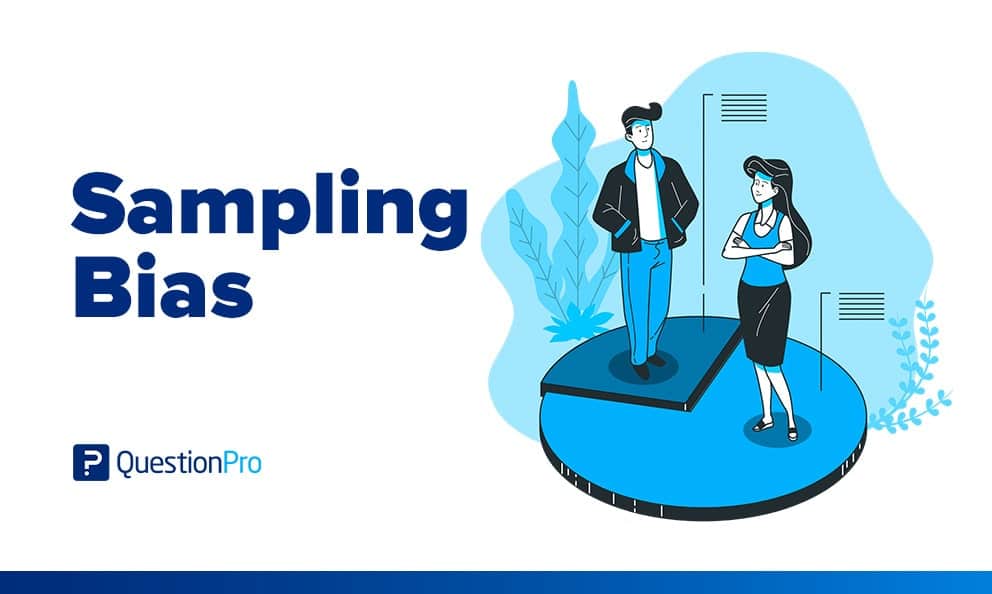
Imagine conducting a research study on the experience of driving a car, but all the participants are exclusively motorcycle users; inevitably, the results will be useless for the research we aimed for. This type of sampling error that occurs when conducting a research study with a poor selection of participants is known as sampling bias, and it can be prevented by always selecting participants at random and from different contexts.
What is Sampling Bias?
Sampling bias, or a biased sample in research, occurs when members of the intended population are selected incorrectly—either because they have a lower or a higher chance of being selected. It is a type of sampling error.
The most popular and easily understandable example of sampling bias is Presidential election voters. If you poll 1000 middle-class, blue-collar voters, the sample will be heavily biased because it won’t be diverse enough to paint the whole picture. It leaves out multiple demographics that are necessary to draw an accurate conclusion.
Causes and Types of Sampling Bias
There are many causes of bias in sampling that researchers need to keep an eye out for. Here are the most common ones:
Undercoverage and Sampling Bias:
Undercoverage is one of the biggest causes of sampling bias because researchers fail to represent the sample accurately. The main reasons for this undercoverage are inadequately representing the population or collecting responses only from readily available respondents by using convenience sampling.
Running national surveys online fall under this risk category of undercoverage because they tend to miss out on the elderly and those with limited or no access to the internet.
A fair representation of the population helps you get accurate results of the survey. But it means that you need to make extra efforts to ensure you do not miss out on different demographics.
Example of undercoverage bias:
Researchers want to understand the effect of a new traffic law in a city, so they conduct a survey sampling via a convenience mall. The study is highly likely to suffer undercoverage from the following groups:
- People who do not like visiting malls
- Those who do not have transportation to the mall
- Those who prefer visiting another mall
Voluntary response bias is also known as self-selection bias where respondents possessing specific characteristics more willingly take part in the research than others. This happens when they have control over the study participation. Here the respondents are not neutral and most tend to lean towards one topic because they identify with it.
Self-selection causes undesirable outcomes in the study and affects its rationality. Voluntary response bias also occurs due to people’s desire to avoid the topic, even though their opinions matter. Thus, the study’s results represent only people who have strong opinions about the topic and exclude the rest, overrepresenting the sample.
Example of self-selection/voluntary bias:
Call-in TV or radio shows are the best examples of voluntary bias, in which only respondents interested in the topic dial in and take part in the study.
Survivorship Bias
Survivorship bias is a common type of sample bias in which the researcher concentrates only on the sample that passes the selection criteria and ignores those who fail to pass. The problem with survivorship bias is that the results are highly optimistic, thus not giving the researcher the whole picture. The opinions of the variables that failed to meet the criteria are ignored, making the results one-sided. A lack of visibility leads to a logical error and skews the end results.
Example of survivorship bias:
Studying business performances in a certain industry may not take into account organizations that have failed and cease to exist now. The results may look positive due to survivorship bias but do not represent the whole industry accurately.
Non-response Bias:
Respondents who refuse to take part in studies and drop out of research cause non-response bias. Non-response occurs due to the inability of a chunk of the target population to take part in the survey because they choose not to. Participation bias happens due to multiple reasons causing a huge bias in studies. Many choose to drop out due to the length or the structure of questions in the study.
Example of non-response bias:
Requesting sensitive information from a sample is one of the main causes of non-response bias. Many respondents may not feel comfortable answering questions about family, income, sexual preferences, drug use and other such personal details leading to bias in responses.
Recall Bias:
Recall bias simply happens when respondents can’t remember things correctly. You can’t do much to mitigate recall bias but can only consider it as a common error in data collection. Recall bias is common in surveys as human memory is imperfect, and they have a selective memory by default. It’s not how good or bad one is at remembering things. The best time to avoid recall bias is to interview respondents when their memory is fresh with the occurrence.
Observer Bias:
Observer bias is caused by researchers when they themselves influence the expectations of the research – either consciously but largely subconsciously. It occurs due to cherry-picking by only looking into a specific statistics group or influencing participants during interviews. A good survey design can mitigate this bias and can be controlled by the researcher.
How to Avoid Sampling Bias
While totally avoiding sampling bias is too much to ask, controlling it to an extent is possible. Here are some tips to avoid sampling bias.
- Define your population and sampling frame.
- Ensure that your target population and sampling frame match
- Keep your survey length short or reasonable
- Make surveys easily accessible
- Follow up
- Convenience sampling is not the best option
- Set your survey goals
- Give respondents a chance to participate equally
The Importance of Stratified Random Sampling
Conducting stratified random sampling is a great way to reduce bias in your studies. It allows researchers to examine the population and create an accurately representative sample.
For example, if your population consists of 5,000 individuals—50% males and 50% females—and 100 people are required to conduct the study, 50 males and 50 females should be chosen to accurately represent the population split. Stratified sampling helps researchers avoid bias by creating awareness of the sampling mix.
Conclusion
One good way to avoid sampling bias is to have a large pool of participants to choose from for your study. A larger to select from gives researchers the chance to sample accurately according to the population. QuestionPro Audience houses millions of double-opted online survey respondents across the globe from which to choose for any type of study. Choose your sampling partner wisely to capture accurate information and run successful research studies.