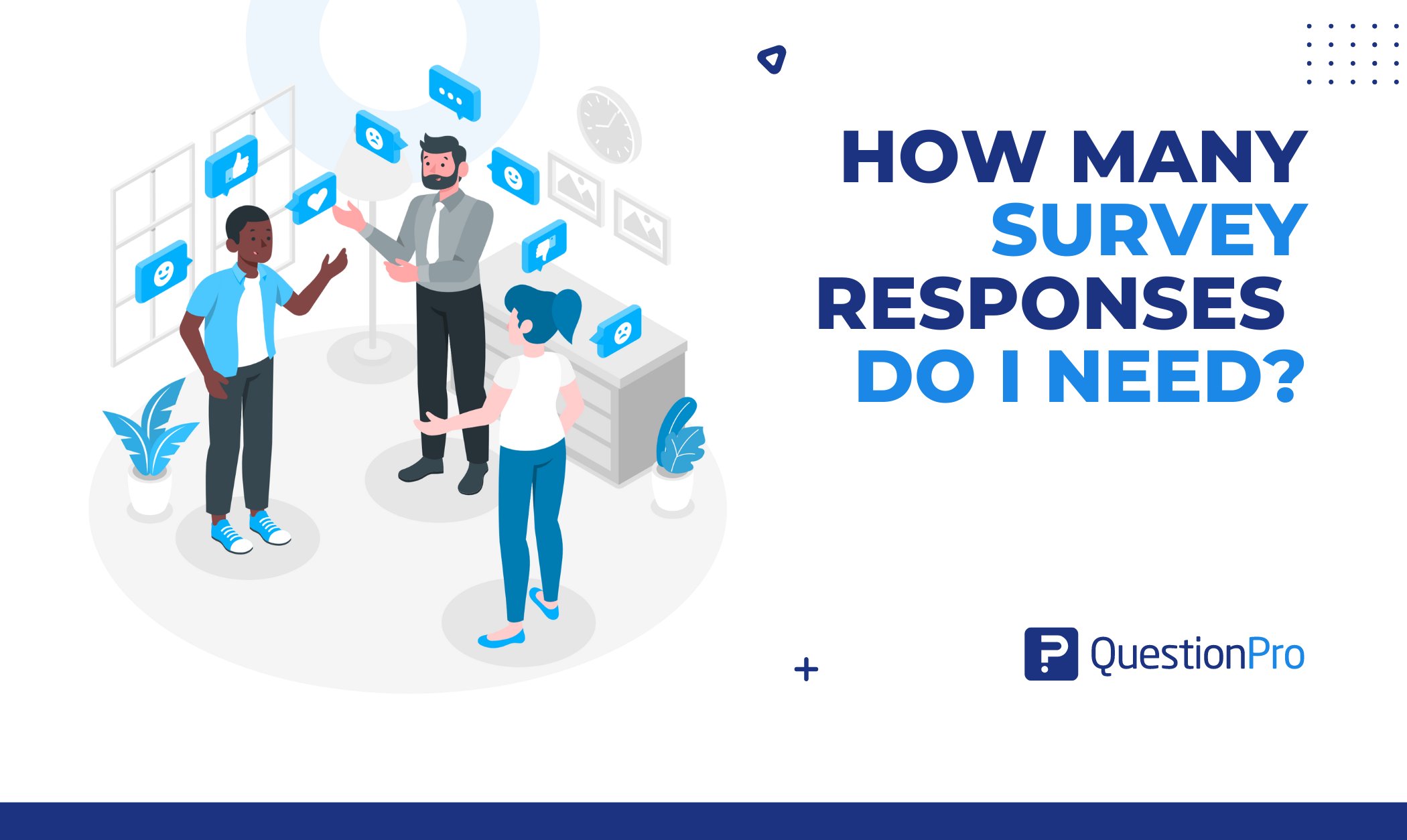
“How many survey responses do I need in survey sample sizes?” Today’s guest post by Gary Angel explores the critical issue of determining the right survey sample size for statistical significance and reliable results. Understanding the factors involved can lead to actionable insights without compromising the user experience.
We just finished our annual web analytics conference – the Web Analytics X Change. The conference is unusual in that it’s all small group discussions with enterprise practitioners. It’s a great format for hearing what’s really bugging people.
As part of the conference, I attended a session focused on survey research and online behavioral integration. One of the most intensely debated topics was online survey sample size.
It’s a big issue because many organizations deploy almost as many different surveys as tags and don’t want to suffer too much from “uncertainty principle syndrome” – damaging the user experience they’re trying to measure.
What are survey sample sizes?
Survey sample size refers to the number of individuals or units selected from the entire population to participate in a survey, and it plays a crucial role in determining a statistically significant sample size while considering the margin of error. It is a critical aspect of survey design and directly impacts the reliability and accuracy of the survey results.
A larger sample size generally leads to more accurate results, as it reduces the impact of random variation or sampling error. Conversely, a smaller sample size can lead to less reliable results and wider confidence intervals.
The formula to calculate the required sample size depends on the survey’s objectives and the analysis type (e.g., estimating proportions, means, comparing groups, etc.). Higher precision, lower variability, and higher confidence will require a larger sample size.
When conducting surveys, researchers often aim to strike a balance between obtaining meaningful results and managing costs and time. A larger sample size may lead to increased expenses and may not always be practical, especially for a smaller population size.
What are survey respondents?
Survey respondents are individuals or participants who provide answers or responses to a survey. When conducting a survey, researchers or surveyors reach out to a specific group of people, known as the target population, and ask them to complete the survey questionnaire or interview.
The success of a survey depends on the willingness of these individuals to participate, which directly influences the average response rate.
Respondents are crucial to the survey process as they represent the source of data that will be used to draw conclusions and insights. The responses provided by the survey participants help researchers understand opinions, attitudes, behaviors, preferences, or other relevant information about the target population.
Survey participants can be chosen using various sampling methods, such as random, stratified, convenience, or purposive, depending on the research objectives and available resources. It is essential to ensure that the survey sample is representative of the target population to draw accurate and valid conclusions.
Survey respondents can participate in different types of surveys, including online surveys, telephone surveys, face-to-face interviews, paper-based surveys, or a combination of these methods.
Their willingness to participate and provide honest and accurate responses greatly influences the quality and validity of the survey results. Researchers often use incentives or other strategies to encourage survey participation and increase response rates.
How many survey responses do I need in survey sample sizes?
Let’s explore the world of survey sample size and gain insights into the art and science of finding the right balance for obtaining actionable survey results.
How many responses are really enough?
There are two schools of thought about sample size – one is that as long as a survey is representative, a relatively small sample size determination is adequate. Perhaps 300-500 respondents can work. The other point of view is that while maintaining a representative sample is essential, the more respondents you have, the better result you get.
This is a big issue because it impacts all sorts of decisions, including the length of your survey, your collection mechanism, and your sampling good survey response rate.
So what’s the right answer?
If you aren’t integrating your survey data with web behavioral data, then a relatively small sample size might be okay (I’d emphasize the might). But if you want to combine behavioral analysis and survey data, then forget a sample of 300 or 500 respondents.
Those numbers simply won’t work. For calculating sample size, our survey sample size calculator can assist you in determining how many participants are required to achieve statistical significance.
Explore further: Sample size calculator.
Let me give you a real-world example showing why that’s true. We’re working with a client that samples approximately 1000 site visitors a month for their satisfaction survey. They asked us to do a study of the impact of using one of two internal search tools on their site on both overall site satisfaction and visit accomplishment.
On this site, search is used in about 10% of visits. Since the site gets more than 10 million visits a month, that still yields a lot of behavior to study – more than 1 million search visits every month. No problem there.
But our sample only captured about 100 respondents who’d used search.
One served about 70% of the queries between the two search tools. So for the second search tool, we had about 30 respondents to deal with. Getting the picture?
For our analysis, we wanted to track visit reason vs. satisfaction vs. outcomes for searchers. With some visit reasons only accounting for about 10% of visits, there were cases where we were supposed to analyze the outcomes for all 3 visitors.
And that’s with a survey size of 1000 and a relatively simple cross-tabulation of visit intent and one fairly common behavior. Sure, we could add lots more months to the picture. But tracking behavior over extended periods adds all sorts of complications to the analysis. The combination of seasonality, site change, and macroeconomic change makes this dangerous. Very few of our client sites remain constant for six months.
If doing behavioral analysis with 1000 survey respondents is challenging, imagine what it would be like with a sample size of 300. Impossible.
So what’s the right answer?
If I had my druthers, I’d recommend that high-volume sites strive for a much higher sample size – something like 15K would be nice monthly.
Is that too much for your user experience to bear? The answer depends on your site volume and take-up and completion rates.
You can’t do much about volume, but if your survey length is impacting your take-ups or completion rates, then I’d be willing to sacrifice a whole bunch of questions to get to the increased size.
On many 30-40 question surveys, we’d only expect to use at most 5-10 of those questions in behavioral analysis. I’d bet even money that your analysts feel that same way and that a heavy majority of questions on many long surveys hardly ever get studied at all.
At some point, you may have to decide: do you want a whole lot of really shallow information, or do you actually want to do analysis on a narrower data set?
So when it comes to behavioral analysis combined with survey integration, the right answer is pretty obvious. A representative sample is essential, but size really does matter. Let yourself get talked into a 300-person sample, and you might as well throw all that work you did to integrate online survey data with behavioral data in the junk pile.
QuestionPro survey software can significantly assist in optimizing survey sample size through its sample size calculation, random sampling capabilities, and diverse survey distribution channels. The software’s various distribution options, including email, social media, and website embedding, help reach a wider audience and increase response rates.
When it comes to behavioral analysis combined with survey integration, the question of “How many survey responses do I need?” becomes crucial. Achieving a representative sample is essential, but size truly matters for meaningful analysis.
QuestionPro survey software offers valuable tools to optimize survey sample sizes, ensuring researchers obtain statistically significant results without unnecessary expenses or compromising data quality.
Frequently Asking Questions (FAQ)
The required number of survey responses depends on factors like desired precision, variability, and confidence level, but a larger sample size generally leads to more reliable results.
The ideal survey sample size varies based on the study’s objectives and available resources; it’s crucial to strike a balance between accuracy and practicality.
Yes, a smaller sample size may result in wider confidence intervals and higher chances of sampling error, reducing the reliability of the findings.