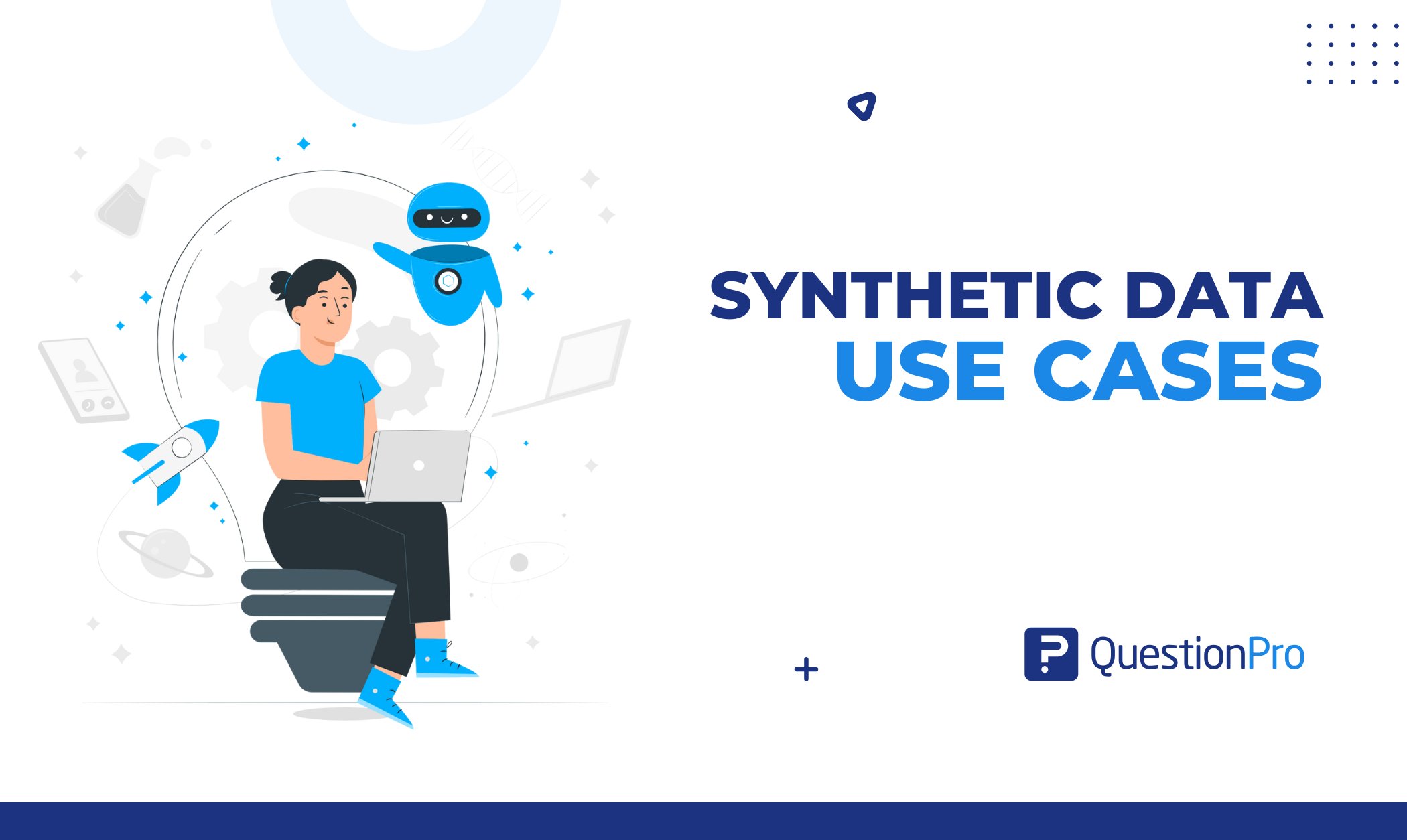
Imagine training a powerful AI model without ever touching real user data — no privacy concerns, no compliance roadblocks, and no costly data collection. You can explore synthetic data use cases from industries such as healthcare, finance, and retail that are rapidly transforming, enabling them to tackle real-world challenges with artificially generated data that’s safe, scalable, and surprisingly accurate.
Synthetic data offers a reliable and secure alternative to real-world data. From catching fraud in banking to training self-driving cars, synthetic data is helping industries solve real problems in safer, faster, and more flexible ways. Let’s explore some use cases of how this “fake” data is making a very real impact across different sectors.
What is Synthetic Data?
Synthetic data behaves like real data. Instead of collecting it from real-life sources, such as customer records, medical data, or bank transactions, it is created using computer programs and smart algorithms.
Think of it like a practice set that mimics real situations. It’s not taken from actual people or events, but it follows the same patterns and rules. This makes it super useful for testing software, training machine learning models, or doing research, without needing to access or risk exposing sensitive real-world information.
Working with real data presents numerous challenges; privacy laws such as GDPR or HIPAA can make it difficult to share or access the data. Synthetic data helps solve that. It provides businesses with a secure and flexible method for generating large datasets without concerns about privacy issues.
Learn More: Synthetic Data Generation Techniques and Considerations
Benefits of Synthetic Data
Synthetic data might be artificial, but the benefits it brings to the table are very real. Here’s why more and more companies are starting to use it:
- Protects Privacy
One of the biggest advantages of synthetic data is that it doesn’t come from real people. That means it doesn’t include any private or sensitive information, making it much safer to use, especially when dealing with strict data protection laws like GDPR or HIPAA.
- Easier to Access and Share
Getting real data can be tricky. Sometimes it’s locked away in secure systems, or companies just can’t share it due to privacy rules. Synthetic data avoids that problem. Since it’s made up but still useful, it can be shared more freely between teams or with outside partners without risking any legal trouble.
- Cost-Effective
Collecting, cleaning, and storing real data can take a lot of time and money. Synthetic data can be created quickly and cheaply. Additionally, you can generate synthetic data as much as you need, whenever you need it.
- Perfect for Testing
Whether you’re testing a new app, website, or machine learning model, you need lots of data to see how things perform. Artificially generated data gives developers realistic test data without needing to wait for real-life scenarios to happen.
- Improves AI Models
Good data is key for training smart AI systems. With synthetic data, you can make sure your AI learns from a wide variety of examples—even ones that are hard to find in the real world. This helps make models more accurate and reliable.
Learn More: Pros and Cons of Synthetic Data and Real Data
Use Cases of Synthetic Data
Synthetic data is like a smart stand-in for real data. It’s created artificially but looks and behaves just like actual data. What makes it so useful is that it helps companies work faster, test better, and stay within privacy rules—all without using real people’s information. Let’s take a look at how different industries are using synthetic data in clever ways.
Financial Services
Banks and financial companies deal with private customer information every day. Sharing or using that real data for testing can be risky or even illegal. That’s where synthetic data helps.
- Fraud Detection: Fraud doesn’t happen often, which makes it hard to train fraud detection systems. Synthetic data can create fake fraud cases to help improve these systems.
- Customer Insights: Instead of using actual customer transactions, companies can use synthetic versions to study behavior and improve services, without exposing anyone’s real details.
Healthcare
Healthcare data is extremely sensitive. Doctors, hospitals, and researchers can’t just use it freely, even when it’s needed to improve treatments.
- Analytics & Research: Synthetic patient records let hospitals and researchers analyze trends or test systems while protecting real patients’ privacy.
- Clinical Trials: In early stages, when real patient data isn’t available yet, synthetic data can be used to model what might happen or guide future research.
Learn More: Synthetic Data in Healthcare
Automotive & Robotics
Testing a robot or self-driving car in the real world can be slow, expensive, or even dangerous. That’s why simulation is key.
Car makers use synthetic driving data to test how cars behave in traffic, bad weather, or emergency situations, without ever hitting the road.
Whether it’s a delivery robot or a warehouse machine, synthetic data helps test its performance in all kinds of environments safely.
Cybersecurity
Security systems need a lot of examples to learn how to spot threats. But collecting that kind of real data is tough.
- Training Security Systems: Synthetic video or image data is used to train cameras and recognition tools to spot people, objects, or suspicious activity.
Social Media Platforms
Social platforms have to constantly watch out for harmful content, like fake news or hate speech. Synthetic content helps test and improve systems that detect and block harmful or misleading posts, without needing to expose users to the real thing.
Developers use synthetic data to test how new features will work without risking user privacy.
Machine Learning & AI
AI needs lots of data to learn, but sometimes the data is rare or hard to label.
- Model Training: Synthetic data can be used to bulk up training datasets, especially when there’s not enough real-world data.
- Rare Events: It’s tough to predict things like fraud or system failures when they happen rarely. Synthetic data helps by creating more examples of those rare events.
HR & Employee Insights
HR departments often can’t share employee data, even within the company. By creating synthetic employee data, companies can safely analyze things like hiring trends or turnover.
New HR tools or algorithms can be tested with synthetic profiles to avoid bias or privacy concerns.
Marketing & Customer Experience
Marketers love data, but privacy laws like GDPR make it harder to use personal information.
- Simulations: Synthetic customer profiles let marketing teams run campaigns, test strategies, and fine-tune ad spending—without needing real user data.
- Customer Journey Testing: They can also model how a customer moves through a website or service and find ways to improve the customer experience.
Software Development & DevOps
Developers often need data to test systems, but getting real data can be slow or risky. Synthetic test data speeds up software testing and keeps it safe.
- Cloud Migration: Moving data to the cloud? Instead of taking risks with real info, companies use synthetic versions to test everything first.
Synthetic data is making a big difference in how industries handle data challenges. It lets companies explore new ideas, build smarter systems, and stay on the right side of privacy rules
Learn More: Synthetic Data Generation Tools & Platforms
Conclusion
We have learned that synthetic data is transforming the way businesses and researchers approach data-driven challenges. It’s not just a backup plan when real data isn’t available—it’s a powerful tool in its own right. By exploring various synthetic data use cases across industries like healthcare, finance, automotive, cybersecurity, marketing, and more, we’ve seen how artificial data can safely mimic real-world scenarios without compromising privacy or security.
While QuestionPro doesn’t create synthetic data itself, it helps set the stage for it. Researchers can use the platform to gather real responses, simulate test data, and organize datasets—all of which can be used later to build synthetic versions. It’s a helpful first step for anyone looking to explore synthetic data in research. Contact QuestionPro for details!
Frequently Asked Questions (FAQs)
Answer: In sectors where privacy is critical, like healthcare and finance, synthetic data allows teams to analyze trends, develop models, and test tools without using sensitive personal information.
Answer: Synthetic data provides diverse and well-labeled datasets that help machine learning models learn better, especially in cases where real data is limited or imbalanced.
Answer: Developers use synthetic data to test applications, systems, and databases without using live or sensitive user data. It speeds up the testing process and ensures privacy compliance.
Answer: Businesses can begin by identifying data-heavy areas with privacy concerns, then use synthetic data tools or platforms to generate artificial datasets for testing, training, or analysis.
Answer: The key benefits of synthetic data include protecting privacy, being cost-effective, offering ease of access and sharing, and enhancing testing capabilities. It allows businesses to work with data that mimics real-world conditions without compromising sensitive information.