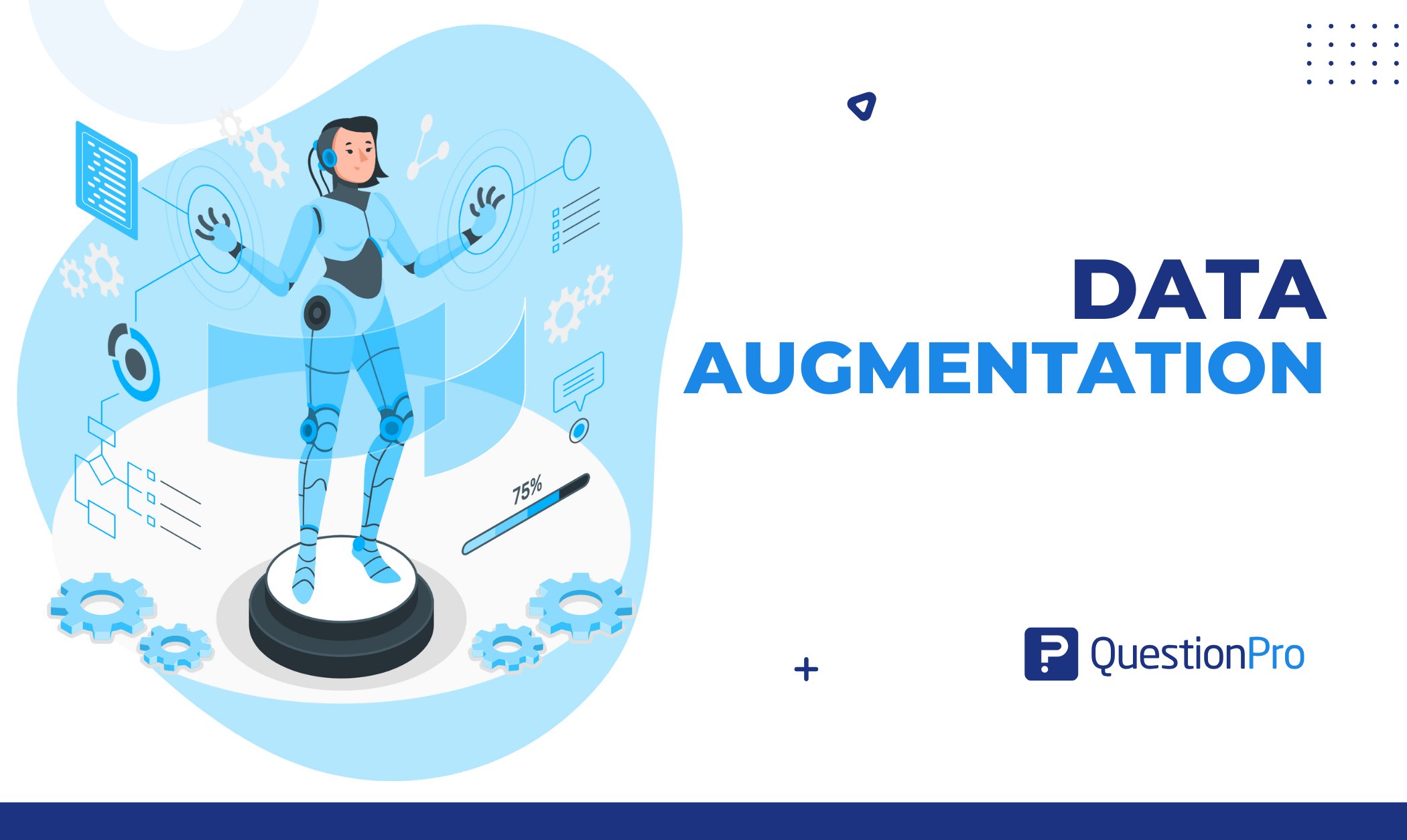
The world is moving forward, and artificial data is one of the key factors that are reshaping the future. As industries adopt AI and machine learning, data augmentation and synthetic datasets are becoming essential for efficient, ethical, and scalable training models.
Unlike real-world data, artificial data can be customized to reduce biases, safeguard privacy, and recreate uncommon situations. Industries like healthcare, finance, and self-driving technology are advancing faster and more responsibly.
With limitless possibilities, it’s clear that the next era of technological advancement will be powered by the data we design, not just the data we collect. We will explore data augmentation to update you about the data era.
What is Data Augmentation?
Data Augmentation is a machine learning and data analysis technique that artificially increases a dataset by creating modified versions of existing data. Instead of collecting new data, it applies transformations like:
- Images: Rotate, flip, blur, and change colors.
- Text: Swap synonyms, paraphrase, translate.
- Audio: Adjust the speed and pitch, and add background noise.
For example, an image can be flipped, rotated, or brightened to create multiple versions of the same image. Similarly, text data can be swapped with synonyms or sentences shuffled to diversify the dataset. This increases the amount of training data and introduces variability, allowing AI models to learn more.
Learn More: Machine Learning Models, Types & Applications
Why Use Data Augmentation Techniques?
If you lack enough training data, data augmentation helps solve the problem. Instead of collecting tons of new data (which can be slow and expensive), we pinch what we already have to make more “fake but realistic” synthetic samples.
This way, models get better without needing endless real-world examples.
Key Benefits:
- Saves time & money: No need to gather tons of new data.
- Prevents overfitting: Helps models work well on new, unseen data.
- Adds variety: It makes AI better handle real-world noise, angles, and changes.
- Balances datasets: Fixes class imbalances (e.g., rare diseases in medical AI).
It’s like giving your AI more “practice scenarios” to avoid choking when things get unpredictable. Augmentation, whether in the form of photos, text, or audio, makes models more innovative and reliable.
Limitations of Augmented Data
While data augmentation is powerful, it’s not a magic fix. Here are its key limitations:
- Overfitting Risk: If augmented data is too similar to the original, models might memorize patterns instead of learning general rules. For example, flipping every image the same way teaches the AI nothing new.
- Unrealistic Outputs: Poorly designed augmentation (e.g., extreme noise in images or nonsensical text swaps) can create “fake” data that misleads models. For example, a blurry tumor scan could confuse a medical AI.
- Domain Gaps: Augmented data might not capture real-world complexity. For example, a self-driving car trained only on sunny-day simulations could fail in heavy rain.
- Privacy Illusions: Synthetic data isn’t always truly anonymous. Re-identification risks exist if patterns mirror real people too closely (e.g., synthetic health records).
- Bias Amplification: If the original data is biased, augmentation can magnify those flaws. For example, facial recognition systems trained on limited skin tone variations perform worse for underrepresented groups.
Augmented data is a tool, not a replacement for thoughtful design and diverse, real-world data. Use it wisely!
Data Augmentation Methods for Research
Data augmentation isn’t just for AI—it’s a powerful tool for enhancing research datasets. Below are methods used for quantitative and qualitative research to simplify the process.
1. Quantitative Data Augmentation
For numerical or structured data:
- Synthetic Data Generation: Use statistical models for synthetic data generation techniques like regression or bootstrapping to create synthetic responses that mirror real survey trends.
- SMOTE (Synthetic Minority Oversampling Technique): Balance imbalanced datasets (e.g., rare customer feedback categories) by creating synthetic minority-class samples.
- Noise Injection: To test model robustness, add slight random variations to numerical data (e.g., survey ratings).
2. Qualitative Data Augmentation
For text, open-ended responses, or thematic data:
- Thematic Expansion: Use NLP tools to paraphrase or expand on open-ended responses (e.g., interview transcripts).
- Scenario Simulation: Create hypothetical scenarios (e.g., “What if” questions) to expand on participant feedback.
- Text Augmentation: Swap synonyms or rephrase sentences in qualitative responses to diversify language patterns.
By blending quantitative rigor with qualitative depth, researchers can overcome data scarcity and build richer, more actionable insights.
Data Augmentation in Quantitative Research
It strengthens quantitative research by improving dataset completeness, balance, and representativeness through imputation, synthetic data generation, and bootstrapping.
- Survey-Based Studies
Data augmentation is key in survey-based research, as it addresses gaps such as missing responses or small sample sizes. Techniques like imputation fill in missing data using statistical methods (e.g., regression models or k-nearest neighbors) so that datasets are complete for analysis.
Replication methods, such as bootstrapping or synthetic respondent generation, expand small survey samples to increase statistical reliability. In public health studies, replicating demographic subgroups reduces non-response bias, leading to more accurate estimates of disease prevalence.
- Small or Imbalanced Datasets
Small or skewed datasets are a big problem in quantitative research. Data augmentation techniques, such as SMOTE (Synthetic Minority Oversampling Technique) or GANs (Generative Adversarial Networks), generate synthetic samples to balance underrepresented classes.
In medical research, rare diseases might have only a handful of cases, and augmenting MRI scans or lab results with synthetic anomalies helps models detect patterns without overfitting. Financial fraud detection systems use augmented transaction records to simulate rare fraudulent behavior.
- Bias Reduction and Generalization Improvements
Augmentation diversifies the training data to reduce bias. For example:- Facial recognition models use varied lighting/ethnic features to improve accuracy across skin tones.
- Synthetic socioeconomic data reduces sampling bias in policy studies.
- Cross-validation shows that augmented models perform 10-20% better on real-world tasks.
Data Augmentation in Qualitative Research
It enhances qualitative research by generating synthetic text, audio, or visuals to deepen analysis, address data scarcity, and uncover hidden patterns. However, it requires careful ethical oversight to preserve authenticity.
- Natural Language Augmentation for Interviews and Transcripts
Qualitative researchers use natural language augmentation to add to text data from interviews, focus groups, or open-ended surveys. Techniques like paraphrasing, synonym replacement, or back-translation (e.g., English to French and back) create linguistic variations while keeping the meaning.
- Ethical Considerations and Interpretability
Augmenting qualitative data raises ethical questions: Do synthetic narratives change participants’ original intent?
In mental health research, even slight changes to interview transcripts can misrepresent lived experiences. Researchers must ensure interpretability by documenting augmentation methods transparently and validating findings against raw data.
- Use in Content Analysis and Thematic Coding
Augmentation adds to textual datasets for deeper analysis:- Synthetic narratives in HIV stigma studies reveal cultural variations in expression.
- Tools like NVivo auto-code augmented text to speed up thematic analysis.
- Be careful: Overaugmentation can create artificial themes and undermine results based on original data.
This balances short bullets for techniques, examples, and lists with paragraphs for context and explanations. Let me know if you need further tweaks!
Data Augmentation in Different Industries
Is data augmentation just for tech labs? Noit’s revolutionizing how researchers approach both quantitative and qualitative studies. Here’s how it’s being used across research methods:
- Healthcare: Expand medical datasets (e.g., generating synthetic X-rays or MRIs) to improve AI-driven disease detection. Creates synthetic patient records for training predictive models without compromising privacy.
- Autonomous Vehicles: Simulates diverse driving conditions (rain, fog) to train perception algorithms with limited real-world data.
- Manufacturing: Augments sensor data to predict equipment failures or generate artificial defects, improving quality-control AI.
- Social Sciences: Generates synthetic interview responses or paraphrased transcripts to identify broader thematic patterns. Augments ethnographic data (e.g., virtual scenarios) to study human behavior in underrepresented contexts.
- Market Research: Expands small focus-group datasets with AI-generated consumer feedback to uncover hidden preferences. Simulates diverse user interactions (e.g., chatbot dialogues) to test qualitative models.
- Content Analysis: Uses NLP to augment text corpora, such as news articles and open-ended surveys, for deeper sentiment or discourse analysis.
From improving statistical reliability in quantitative studies to uncovering nuanced insights in qualitative work, data augmentation helps researchers overcome data scarcity, bias, and ethical constraints without sacrificing rigor.
Learn More: The Impact Of Synthetic Data On Modern Research.
Augmented Data Vs. Synthetic Data
Here’s a clear comparison chart between Augmented Data and Synthetic Data in research contexts:
Feature | Augmented Data | Synthetic Data |
Definition | Modified or expanded versions of real data. | Artificially generated data that mimics real-world patterns. |
Purpose | Enhance existing datasets without losing original meaning. | Replace or supplement scarce/private real data. |
Quantitative Use | Bootstrapping survey samples. | Generate synthetic clinical trial data. |
Qualitative Use | Paraphrased interview transcripts. | AI-generated open-ended survey responses. |
Pros | Preserves core data integrity. | Solves data scarcity. |
Cons | Risk of overfitting if overused. | May lack realism. |
Pro Tips:
- Use augmented data to strengthen existing datasets.
- Use a synthetic dataset to replace missing or sensitive data.
How a Combination of QuestionPro Research Suite and Data Augmentation Works for Researchers!
QuestionPro’s Research suite and data augmentation techniques help researchers build bigger datasets and train more accurate AI/ML models, especially in computer vision and deep learning. Here’s how they work together:
1. Enriching Training Datasets
QuestionPro is the starting point for collecting high-quality input data, including text responses, images, or behavioral data.
Researchers can use techniques like paraphrasing or synonym replacement when working with text data from surveys to generate more variations while preserving the original meaning.
2. Improving Model Performance
Combining QuestionPro’s data collection and augmentation techniques allows researchers to build more robust training datasets for deep neural networks.
Advanced data augmentation techniques, including generative adversarial networks (GANs), can generate synthetic data with the same statistical properties as real data while maintaining its privacy. This is particularly useful for training object detection models and other deep learning applications where diverse data is key for model generalization.
3. Applications in Research
Combining QuestionPro Research Suite and data augmentation in market research allows researchers to test pricing models by combining real survey data with synthetic demographic variations.
Social scientists can use these techniques to generate synthetic interview transcripts for testing and refining thematic coding frameworks.
QuestionPro is the foundation of high-quality input data; data augmentation expands and enriches this data for further analysis. Together, they are the complete solution for researchers working with traditional statistical models and AI systems.
Conclusion
Combining QuestionPro (for robust data collection) with augmentation methods (for dataset expansion) is a game-changer for healthcare to the social sciences.
Researchers can train more accurate AI models, find hidden patterns, and simulate rare scenarios without compromising data integrity or privacy.
As technology advances, the future of research is in intelligent data design, not just collection. Strategically using augmented and synthetic data allows researchers to go further, generalize more, and innovate across fields.
Frequently Asked Questions(FAQs)
Answer: Data Augmentation is a machine learning and data analysis technique that artificially increases a dataset by creating modified versions of existing data.
Answer: Data augmentation enhances quantitative research by improving dataset quality and reliability. It addresses missing data through imputation techniques, expands small samples via bootstrapping or synthetic respondent generation, and balances skewed datasets using methods like SMOTE or GANs.
Answer: The technique risks overfitting if augmented data lacks diversity. It can produce unrealistic outputs that mislead models and may fail to capture real-world complexity, like weather variations for autonomous vehicles. Additionally, synthetic data might preserve privacy risks if it too closely mirrors real individuals, and it can amplify existing biases in the original dataset.
Answer: No, augmented data and synthetic data are not the same. Augmented data starts with real datasets and applies modifications to create expanded but derivative versions. Synthetic data is entirely artificially generated to mimic real-world patterns, often used when original data is limited or sensitive.