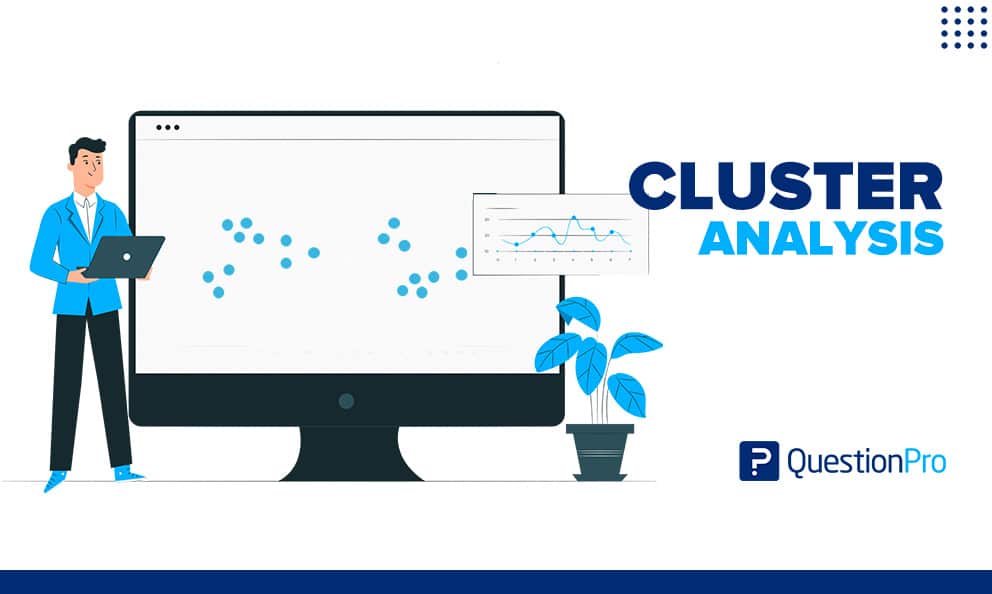
Data is imperative for brands and organizations to derive inferences and draw conclusions into the minds of customers. Cluster analysis is a critical component of data analysis in market research that aids brands with deriving trends, identifying groups among various demographics of customers, purchase behaviors, likes and dislikes, and more.
This analysis method in the market research process provides insights to bucket information into smaller groups that help understand how different groups of individuals behave under similar circumstances. Various organizations and researchers can qualify clusters into varied categories depending on pre-defined criteria of what makes sense of a cluster, but the underlying data analysis theme is similar.
What is cluster analysis?
Cluster analysis is a statistical method in research that allows researchers to bucket or group a set of objects into small but distinct clusters that differ in characteristics from other such different clusters. The underlying theme in exploratory data analysis helps brands, organizations, and researchers derive insights from visual data to spot trends and validate hypotheses and explicit assumptions.
This analysis method in research is commonly based on statistical data analysis used in varied fields, including pattern recognition, machine learning, insights management in market research, data scrubbing, bioinformatics, and more.
The objective of cluster analysis is to find groups of objects with distinct behavioral changes but where the underlying characteristics and the things are in the same control group. An excellent example of this research method is banks using qualitative and quantitative data to plot trends in claims processing among clients. Using cluster analysis helps them conclude fraudulent claims and better understand consumer behavior.
Discover a wealth of insights in our latest article, showcasing diverse examples of qualitative data in education.
Cluster Analysis Methods
Cluster analysis helps researchers and statisticians to make a more profound sense of data and make better decisions. While the data can be a part of qualitative research or quantitative research, data analysis is still conducted in a research platform where the data is plotted on a graph. However, as mentioned above, various cluster analysis methods are used to suit research needs.
However, it is essential to note that the clustering method needs to be chosen experimentally unless there is mathematical reasoning to go with a specific manner. Let us look at the most commonly used cluster analysis methods.
Hierarchical clustering or connectivity-based clustering analysis
Hierarchical clustering or connectivity-based clustering analysis is the most commonly used method in cluster analysis. In this method, data that showcase similar components are grouped to form a cluster.
These clusters are then correlated to other sets that show identical properties to form other clusters. The central premise of this method in survey research is that objects closer are much more related than objects farther apart.
The other method in hierarchical clustering is the divisive method, where you start with a set of data and then divide them into smaller clusters of similar information. In this method, linkage criteria between clusters are better defined to understand the distance between clusters and their relation. It is important to note that there is no single data partitioning in this analysis model.
Centroid-based clustering
In this clustering method, clusters are formed but are defined by a single central vector point. Using the K-means method clustering algorithm, a central point is found on the axis with a defined objective. Then smaller clusters are connected to this central such that the distance between the clusters and this central point is minimized.
A drawback of this cluster analysis technique is that the number of clusters, k-clusters is to be defined right at the outset, limiting data analysis and representation.
Distribution-based clustering
The distribution-based clustering analysis method groups data into objects of the same distribution. This method is the most widely used statistical analysis method. This method’s distinct characteristic is simple random sampling to collect sample objects from a distribution.
This model works best when there is a need to display a correlation between attributes and objects. However, the drawback of this model is that since objects are grouped based on predefined attributes, there could be an element of bias in the clustering since each object must match a distribution.
Density-based clustering
The density-based clustering method is the fourth commonly used cluster analysis technique, where clusters are defined based on density compared to the overall data set. The objects in the sparse areas are noise and border points, as they typically separate clusters on the graphical representation.
DBSCAN is the most commonly used density-based clustering method. However, a drawback of this method is that a drop in density is required to showcase the difference between two clusters, which often feels unnatural.
Cluster Analysis Examples
Cluster analysis is a definite benefit, and it is widely used across industries, functionalities, and the research field. To better depict the usefulness of cluster analysis in research, let us look at the bottom two examples.
Cluster analysis in retail marketing
Brands traditionally use cluster analysis to make sense of purchase behavior research and trends by using demographic segmentation among their customer base. A few factors usually considered are geographical location, sex, age, annual family income, etc.
These parameters highlight how different consumer groups make other purchase decisions; hence, retail giants use this data to draw parallels on how to market to such audiences. This also helps in maximizing the ROI on spending while reducing customer churn.
Cluster analysis in sports sciences
Another everyday use case of cluster analysis is in the field of sports. Data scientists, researchers, doctors, team management, scouts, etc., look at how similar players fare in different scenarios and how effective they are in their sport. Players are bucketed into body type, age, position, and similar criteria to check their effectiveness.
Cluster Analysis with QuestionPro
Looking at the correct data and analyzing it is highly beneficial for researchers and brands. Using a mature research platform like QuestionPro allows you to collect research data and helps you run advanced analysis within the tool to give you the insights that matter.
Leveraging QuestionPro, it is possible to understand your customers and other research objects better and quickly make decisions that matter. Leverage the power of the enterprise-grade research suite today!