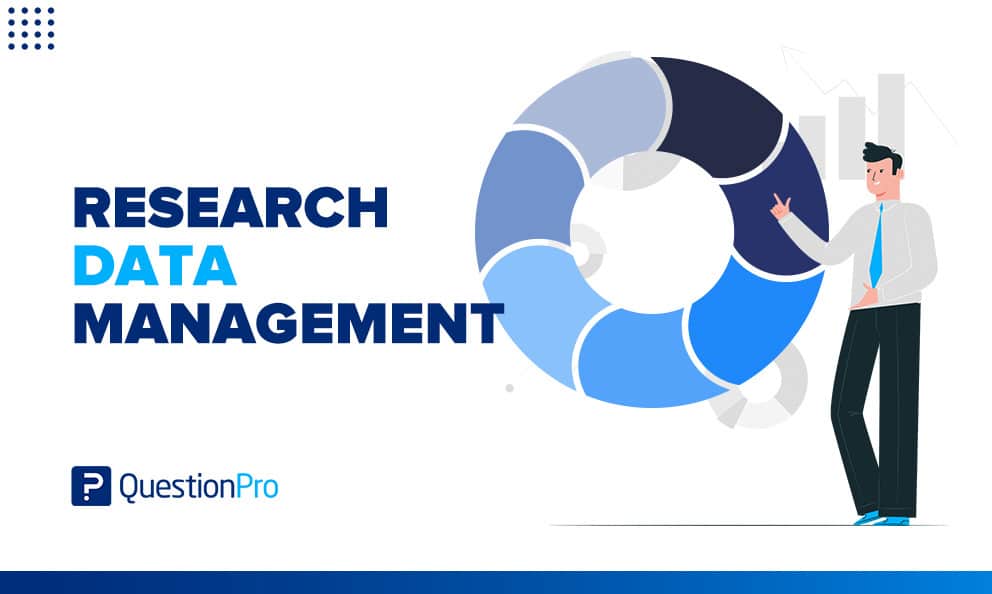
With the proliferation of data and the need for agile and fast insights, research teams, researchers, and organizations worldwide need quicker access to the correct data, which is possible with research data management.
Research is conducted for various reasons, including academic research, pricing research, brand tracking, monitoring, competitive research, ad testing, longitudinal tracking, product and service upgrades, customer satisfaction, etc. The data generated during the research process is diverse and vast.
LEARN ABOUT: Pricing Research
Accessing the correct data in the proper format allows for the data democratization of insights, reduces the silo in research, and eliminates tribal knowledge with mature insights management tools such as InsightsHub. A recent Statista report stated that the global revenue from the market research industry exceeded $74.6 billion in 2021, and that number is only expected to grow.
With data at this scale, it is imperative to have systems in place to make the most of the data in the shortest possible time, and that’s where research data management comes in.
LEARN ABOUT: Research Process Steps
What is research data management?
Research data management (or RDM) is the action of vigorously organizing, storing, and preserving data during the market research process. RDM covers the data lifecycle from planning through to people, process and technology, and long-term monitoring and access to the data. This is a continuous ongoing cycle during the process of data.
Research data comes in many forms and types, especially with various types of research that include qualitative and quantitative research. This means the data also can be of multiple scales and types. RDM helps to classify, categorize and store this information in a way that’s easy to understand, reference, and draw inferences from.
Data management in research follows the fundamentals of the data life cycle, which are critical steps in research data management as listed below:
- Plan: The plan includes involving stakeholders, defining processes, picking the tools, defining data owners, and how the data is shared.
- Create: Researchers and research teams create the data in the form of data collection techniques defined by projects and then put together this data in structured formats with relevant tags and meta-descriptions.
- Process: This raw data is then converted into digital data in the organization’s structure. The information is cleaned, scrubbed, and structured to eliminate time for insights.
- Analyze: A critical component of RDM is analyzing research data to derive actionable insights from the data that has been collected. This data can then be structured into consumable data.
- Preserve: The raw and analyzed data is then preserved in formats defined in the earlier process to maintain the quality of information.
- Share: Distribution of insights to the right stakeholders in role-based access control is required so that the insights are then actioned upon to match business and research goals.
- Reuse: With the correct metadata, tagging and categorization, it is possible to reuse research data to draw correlations, increase ROI and reduce time to research studies.
All the above steps aid in innovative research data management and are critical to market research and insights management success.
LEARN ABOUT: Action Research
Research data management benefits
Following good research data management practices has multiple benefits. Some of the most important ones, however, are:
-
Maintain the sanctity of data and increase accountability
An essential benefit of RDM is that it allows the ability to maintain the sanctity of the collected data and increases accountability across the board and for all stakeholders. There is absolute transparency in how the information is collected, stored, tracked, shared, and more, along with the additional benefits of following data storage compliances and regulations. Defined processes also lead to lesser ambiguity about stakeholders and data owners and how it is to be monitored.
-
Eliminate tribal knowledge
Since there is an expectation that data is to be managed in a specific manner, everyone follows the same process. This eliminates tribal knowledge when people either leave organizations or new members come into the fold. It also ensures that stakeholders and researchers from cross-functional teams can lean in on past data to make inferences.
-
Democratize insights
Insights are powerful when they’re accessible to the right teams at the right time. With research data management, there is an assurance that even if it is role-based access, a larger pool of members has access to the data regardless of the research design and type. There is greater visibility in the tools used; the audience reached, granular, and analyzed data, which in turn helps to democratize insights.
-
Enable longitudinal monitoring and quick turnaround studies
No matter what type of research is being conducted, RDM allows users to either draw comparisons from past studies or use the past data to validate or disprove hypotheses. With easy access to data, there is also the ability to conduct longitudinal studies or quick turnaround studies by leaning on past, structured data.
-
Avoid duplication of efforts and research
Brands and organizations use a market research platform to conduct research studies. With a data management software plan in place, you can avoid redoing the same or similar research and reducing the research’s geo-location borders and validity. It also helps reduce duplication of efforts as you do not have to start from scratch.
-
Reduce time and increase the ROI of research
With easy access to structured data and insights, the time to insights is reduced as there is a reduction of duplicity in research projects. There’s also the scope of inferences to past data and data across demographics and regions. There’s scope to do more with less. All of the above aids in increasing the ROI of research as the effort spent is lesser, but the output is higher, which aids with continuous discovery.
Research data management examples
As seen above, there is research data management is forming an integral part of organizations and research teams to derive the most out of their research processes.
To illustrate this better with an example, consider a retail giant with a presence in many countries. To stay above the competition, create sticky customers, and to constantly co-create with customers, multiple research techniques and methods are used continuously.
This research helps to understand the brand value, consumer behavior, pricing sensitivity, product upgrades, customer satisfaction, etc. By implementing a solid RDM strategy, the brand can lean on past and existing studies, both qualitative and quantitative, to draw inferences about pricing preferences across markets, seasonal launches, what works in different needs, perception of brand vs. their competitors, etc. There is also the ability to look at historical data to manage inventory or budget for marketing spending.
When done well, a good research data management strategy and the right knowledge discovery tools can work wonders for brands and organizations alike.
Get the most out of your research data management
With QuestionPro, you have access to the most mature market research platform and tool that helps you collect and analyze the insights that matter the most. By leveraging InsightsHub, the unified hub for data management, you can leverage the consolidated platform to organize, explore, search, and discover your research data in one organized repository.
LEARN ABOUT: Customer data management