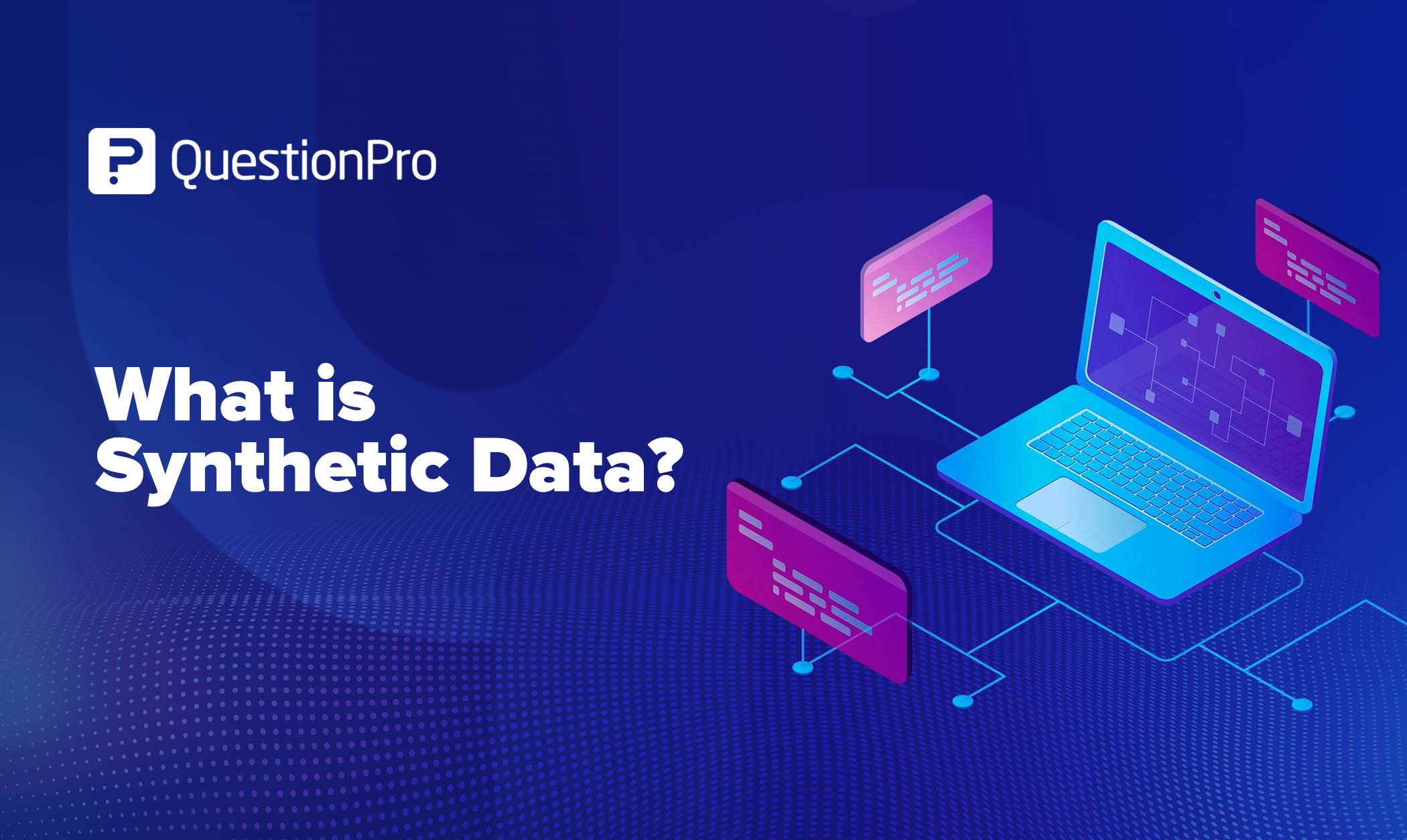
Synthetic data isn’t just another tool in the AI toolbox — it’s changing the way we train models, fuel research, and even challenge long-held assumptions about how much real-world data we actually need.
This concept often gets lost in the sea of tech buzzwords that surround the world of Artificial Intelligence these days. However, if you’ve made it to this article, chances are you’re genuinely interested in learning more about what synthetic data is, how it’s created, its validity, and the different types available.
Exploring this topic will not only help you stay ahead of the curve but also give you a clearer understanding of why synthetic data is quietly reshaping the way industries tackle research challenges.
What is Synthetic Data?
Synthetic data is artificially generated data replicating real-world data’s qualities and statistical properties. The big difference and also a great advantage of this type of data is that it does not contain any actual information from real people or sources. It’s like copying the patterns, trends, and other features found in real data but without any real information.
So you’re probably wondering where this data comes from? Synthetic Data is created using various algorithms, models, or simulations to recreate the patterns, distributions, and correlations in actual data. The goal is to generate data that matches the statistical qualities and relationships in the original data while avoiding revealing individual identities or sensitive details.
When you use this artificially generated data, you benefit from not dealing with the limits of using regulated or sensitive data. You can customize the data to fulfill specific requirements that are impossible to meet with real data. These synthetic data sets are mainly used for quality assurance and software testing.
However, you should be aware that this data also has downsides. Replicating the complexity of the original data may result in discrepancies. It should be noted that this artificially generated data cannot wholly replace genuine data, as reliable data is still required to create relevant findings.
The concept is relatively new, and while it might seem complicated at first, the truth is — it’s easier to understand than you might think, especially when explained by the right experts. That’s why at QuestionPro, we’ve brought together Ken Fleetwood and Chris Robinson for our latest webinar, where they break down everything you need to know about Synthetic Data.
If you want a clear overview of this emerging methodology, don’t miss the chance to check it out below!
Synthetic Data Benefits
Synthetic data provides several advantages over data analysis and machine learning, making it a vital tool in your toolbox.
By creating data that reflects the statistical features of real-world data, you can open up new opportunities while maintaining privacy, cooperation, and the development of robust models.
There are currently many applications for this type of data, and over time, new and improved ways to leverage the benefits of this new type of information are being discovered. Below, we list some use cases to give you an idea of the scope and potential of this methodology.
Avoid Privacy Concerns
Assume you’re working with sensitive data, such as medical records, personal identifiers, or financial information. Synthetic data will act as a shield, allowing you to extract useful insights without exposing individuals’ privacy.
You can maintain confidentiality while conducting critical analysis by generating statistically similar data that is not identifiable to real people.
Data Sharing and Simulated Collaboration
This artificially generated data shines as a solution in situations when data exchange presents challenges like legal limits, proprietary issues, or cross-border legislation.
Using synthetically generated datasets, you may stimulate collaboration without revealing sensitive information. Researchers, institutions, and companies can exchange vital knowledge without the typical restrictions.
Model Development and Testing
You can develop accurate, efficient models with synthetically generated data. Consider it your testing space. You may effectively fine-tune your models by testing them on carefully prepared synthetic test data that replicates real-world distributions.
This artificial data will help you detect problems early. It prevents overfitting and ensures the accuracy of your models before deploying them in real-world scenarios.
Real-World Use Cases
Synthetic data finds application in a diverse range of real-world scenarios, offering solutions to various challenges across different domains. Here are some notable use cases where the artificial data proves its value:
- Healthcare and Medical Research: Synthetic data in healthcare and medical studies is used to distribute and evaluate medical data without compromising patient privacy. Simulating patient records, medical imaging, and genetic data allows researchers to create and test algorithms without exposing sensitive data.
- Financial Analysis: This artificial data tests investment strategies, risk management models, and trading algorithms. Analysts can test alternative scenarios and make informed conclusions. They can do so without using sensitive financial data by recreating market behaviors and financial data.
- Fraud detection: Without revealing client data, financial institutions can develop synthetic transaction data that simulates fraud. This helps develop and improve fraud detection systems.
- Social Sciences: Without breaching privacy, social scientists can analyze trends, habits, and social interactions. Researchers can examine and model human behavior, perform surveys, and simulate social settings to understand societal dynamics.
- Online Privacy Protection: Fake data can preserve consumers’ privacy in privacy-sensitive applications like online advertising or customized recommendation systems. Advertisers and platforms can optimize ad targeting and user experiences using synthetic user profiles and behaviors to maintain user anonymity.
Types of Synthetic Data
Synthetic data offers many methods to suit your needs. These techniques protect sensitive data while retaining important statistical insights from your original data. Synthetic data can be divided into three types, each with its own purpose and benefits.
1. Fully Synthetic Data
This artificial data is entirely made up and contains no original information. In this scenario, as the data generator, you would typically estimate the density function parameters of the features present in the real world data. Then, using the projected density functions as a guide, privacy-protected sequences are created randomly for each characteristic.
Let’s say you decide to replace a small number of real data attributes with artificial ones. The protected sequences for these features align with the other properties found in the actual data. Because of this alignment, the protected and real sequences can be ranked similarly.
2. Partially Synthetic Data
This artificial data comes into play when it comes to protecting privacy while keeping the integrity of your data. Here, selected sensitive feature values that offer a high risk of disclosure are replaced with synthetic alternatives.
To create this data, approaches like multiple imputation and model-based methods are used. These methods can also be used to impute missing values from your actual data. The goal is to keep your data’s structure intact while preserving your privacy.
3. Hybrid Synthetic Data
This artificial data emerges as a formidable alternative for achieving a well-balanced compromise between privacy and utility. A hybrid dataset is created by mixing actual and artificially created data aspects.
A closely related record from the synthetic data vault is chosen for each random record in your real data. This method combines the advantages of totally synthetic and partially artificial data, finding a compromise between excellent privacy preservation and data value.
However, because of the combination of real and synthetic elements, this method can require more memory and processing time.
Synthetic Data Generation Methods
You can explore a range of synthetic data-generating methods, each offering an individual technique for producing data that accurately reflects the complexities of the actual world.
These techniques allow you to produce synthetic datasets that preserve the statistical foundations of real data while opening up fresh possibilities for exploration. Let’s explore these approaches:
Statistical Distribution
In this method, you draw numbers from the distribution by studying real statistical distributions and reproducing similar data. When real world data is unavailable, you can use this factual data.
Data scientists can construct a random dataset if they understand real data’s statistical distribution. Normal, chi-square, exponential, and other distributions can do this. The accuracy of the trained model is strongly dependent on the data scientist’s expertise with this method.
Agent-Based Modeling
This method allows you to design a model that will explain observed behavior and will produce random data using the same model. This is the process of fitting actual data to a known data distribution. This technology can be used by businesses to create AI generated synthetic data.
Other machine-learning approaches can also be employed to customize the distributions. However, when the data scientists wish to forecast the future, the decision tree will overfit due to its simplicity and reach full depth.
Generative Adversarial Networks (GANs)
In this generative model, two neural networks collaborate to generate manufactured, but possibly valid, data points. One of these neural networks acts as a creator, generating synthetic data points. On the other hand, the other network serves as a judge, learning how to differentiate between created fake samples and actual ones.
GANs may be challenging to train and computationally expensive, but the return is well worth it. With GANs, you can generate data that accurately reflects reality.
Variational Autoencoders (VAEs)
It’s a method without supervision that can learn the distribution of your original dataset. It can generate artificial data via a two-step transformation process known as an encoded-decoded architecture.
The VAE model produces a reconstruction error, which can be reduced through iterative training sessions. By using VAE, you can obtain a tool that allows you to generate data that closely resembles the distribution of your real dataset.
Challenges and Considerations
When dealing with synthetic data, be prepared to face several challenges and limits that can have an impact on its effectiveness and applicability:
- Accuracy of Data Distribution: Replicating the precise distribution of real-world data can be difficult, potentially leading to mistakes in generated artificial data.
- Maintaining Correlations: It is difficult to maintain complicated correlations and dependencies between variables, which impacts the reliability of the synthetic data.
- Generalization to Real Data: Models trained on artificial data may not perform as well as expected on real-world data, needing thorough validation.
- Privacy vs. Utility: Finding an acceptable balance between privacy protection and data utility can be difficult, as severe anonymization can compromise the data’s representativeness.
- Validation and Quality Assurance: Because there is no ground truth, thorough validation procedures are required to ensure the quality and dependability of synthetic information.
- Ethical and legal considerations: Mishandling artificial data can raise ethical problems and legal consequences, which highlights the importance of suitable usage agreements.
Validation and Evaluation
When working with artificial data, thorough validation and evaluation are required to ensure its quality, applicability, and reliability. Here’s how to effectively validate and evaluate this fake data:
Measuring Data Quality
- Comparing Descriptive Statistics: To verify alignment, compare the statistical attributes of this artificial data to real data (e.g., mean, variance, distribution).
- Visual Inspection: Visually identify discrepancies and variances by plotting synthetic data against real data.
- Outlier Detection: Look for outliers that could impact artificial data quality and model performance.
Ensuring Utility and Validity
- Alignment of Use Cases: Determine whether the artificial data meets the requirements of your specific use case or research issue.
- Model Impact: Train machine learning models and then evaluate their value on original data.
- Domain Expertise: Include domain experts in the validation process to ensure that the artificial data captures essential domain-specific properties.
Benchmarking Synthetic Data
- Comparison to Ground Truth: If accessible, compare generated data to ground truth data to determine its accuracy.
- Model Performance: Compare the performance of machine learning models trained on synthetic data against models trained on real data.
- Sensitivity Analysis: Determine the sensitivity of results to changes in data parameters and creation methods.
Continuous Development
- Feedback Loop: Continuously improve and adjust data depending on validation and evaluation feedback.
- Incremental Changes: Adjust generation processes gradually to increase data quality and alignment.
Future Trends in Synthetic Data
As you look ahead, several exciting trends are shaping the future of synthetic data, impacting how you generate and use data for various purposes:
- Customization for Your Needs: In the future, technologies will be available. These will let you customize synthetic data to particular industries or your own needs, and this customization will increase relevance.
- The Rise of Data Augmentation: Synthetic information will progressively complement real datasets through data augmentation. This will improve model resilience and performance.
- Ethical and bias considerations: Tools for detecting and mitigating biases will emerge, which will support fairness in AI applications.
- Standardization and Transparency: To improve trustworthiness and openness, it’s important to look out for initiatives aimed at standardizing the data methods. Additionally, look for efforts to develop benchmark datasets.
- Transfer Learning Integration: Synthetic information may be crucial in pretraining models on simulated data. This can decrease the need for large, original real data for specific tasks.
Want to learn more about how you can generate your synthetic data in minutes and a simplified manner? We invite you to read our extensive guide on: Best Synthetic Data Generation Tools.
Conclusion
The potential of synthetic data is becoming clearer. By strategically adding it to your toolkit, you can empower yourself to face obstacles creatively and precisely.
Data scientists can utilize synthetic data to its maximum potential. Their expertise can lead the way for data privacy protection. It can also enrich model development with diverse and adaptable datasets and foster collaboration that transcends conventional boundaries.
QuestionPro can be a significant resource in realizing the possibilities of synthetic data. The platform empowers you to take full advantage of the benefits of synthetic data for your research, analysis, and decision-making processes with our extensive range of tools and features.
Use QuestionPro’s survey design software to collect accurate data from your target audience. This genuine data serves as the foundation for producing significant fake data. You can use QuestionPro to convert raw survey responses into structured datasets. This results in a smooth transition from raw data to synthesized information.
With the help of QuestionPro’s complete tools and experience, you can confidently enter the future of data science.