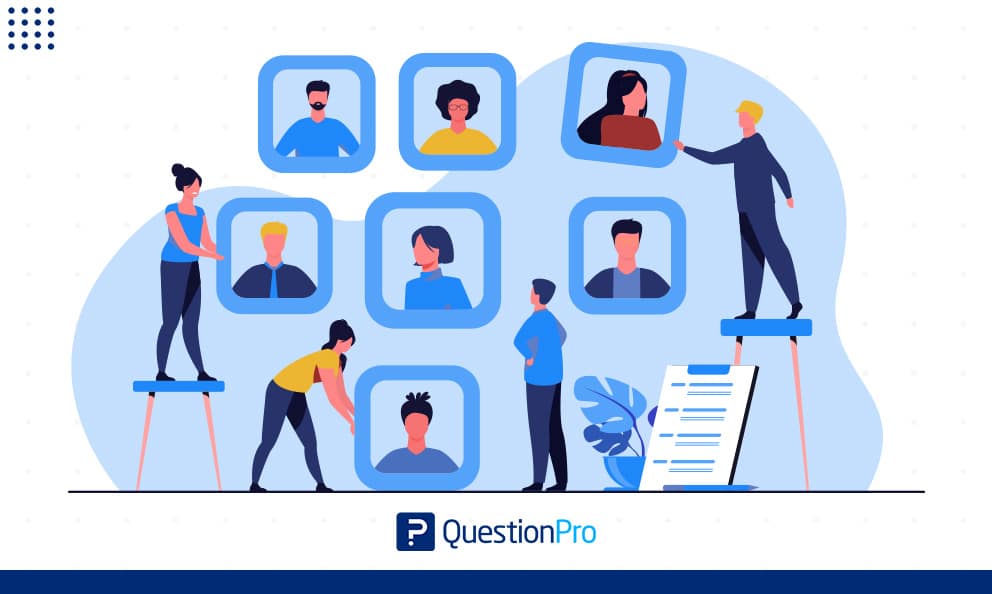
Stratified sampling is a sampling procedure in which the target population is separated into unique, homogeneous segments (strata), and then a simple random sample is selected from each segment (stratum). The selected samples from the various strata are combined into a single sample. This sampling procedure is sometimes referred to as “occasional fee sampling.” Read below about some of the considerations to remember for the best capture.
Stratified sampling is one of the types of probabilistic sampling that we can use. I invite you to continue reading to learn more about its weaknesses and strengths.
What is stratified sampling?
Stratified sampling is a way to get a representative sample from a community that researchers have split into groups (called “strata”) that are similar to each other. Researchers use the stratified sampling technique to make sure that their sample includes members of certain classes. It also helps them make accurate estimates of what each group is like.
This method is used in numerous research projects to learn more about the differences between subpopulations. This method is a type of probability sampling called “stratified random sampling.”
In the first step of the stratified sampling method, researchers divide a diverse community into mostly the same groups. These groups are called strata, which is the plural of the stratum.
Then, they take random samples from each group (called a “stratum”) and put them all together to make a full sample that is representative of the whole. Learn more about representative groups. Quota sampling is when researchers choose subjects from the groups in a not random way.
Strata are smaller groups of people who are mostly the same as each other compared to the whole community. Researchers can divide groups into classes based on income, gender, race, and many other factors.
For example, if your research question asks you to compare how things turn out for people with different incomes, you might divide the groups by income. There should only be one stratum for the whole community.
Selection steps for a stratified sampling survey
There are eight main steps in selecting a stratified random sample:
Step 1: Define the target population
Clearly define the population you want to study, specifying the characteristics and boundaries of the population.
Step 2: Identify stratification variables
Determine the key characteristics or variables that are important for dividing the population into meaningful subgroups or strata. These variables should be relevant to the research objectives and should help in creating distinct groups within the population.
Step 3: Determine the number of strata
Decide how many strata to create based on the identified stratification variables. The number of strata should be determined by the variability within the population and the level of precision required for the study.
Step 4: Develop or identify a sampling frame
Obtain or create a sampling frame that includes information on the stratification variables for each item in the target population. A sampling frame is a list or database that represents the population from which the sample will be selected.
Step 5: Evaluate the sampling frame
Assess the sampling frame for any issues such as undercover (missing elements from the population), overcover (duplicated or irrelevant elements), multiple listings, or clustering. Make adjustments to the sampling frame as necessary to ensure its accuracy and appropriateness for sampling.
Step 6: Divide the sampling frame into strata
Divide the sampling frame into separate strata based on the identified stratification variables. Each element in the population should be assigned to one and only one stratum, ensuring that the strata are mutually exclusive and collectively exhaustive.
Step 7: Determine the sample size for each stratum
Decide on the desired sample size for the overall survey and the sample size for each stratum. The sample size for each stratum should be determined based on the proportion of the population that belongs to that stratum. This ensures that each stratum is represented appropriately in the final sample.
Step 8: Randomly select samples from each stratum
Randomly select the specified number of items from each stratum using random sampling techniques. This ensures that each element in the population has an equal chance of being selected and allows for representative sampling within each stratum.
Subtypes of stratified sampling
There are two main subtypes of stratified sampling: Proportionate and disproportionate sampling.
Proportionate Stratified Sampling
In proportionate stratification, the number of items assigned to various strata is proportionate to the strata’s representation of the target population. That is, the final sample size drawn from each stratum is proportionate to the relative size of that stratum of the target population.
The sampling fraction is applied to each stratum, giving each population element an equal opportunity to be selected. The resulting sample is self-weighted. This sampling technique is used when the research aims to estimate population parameters.
The researcher often wishes not only to estimate population parameters but also to do detailed analysis within a relatively small stratum and/or to compare strata with each other. Proportionate stratified random sampling may not result in some of the strata of this type of analysis.
Taking the example described in our table, it would not be possible to carry out a detailed analysis of the elements in zone 2 since only 12 of the elements are found in the sample.
Furthermore, the comparison of the elements of Zone 2 with the other zones would be doubtful.
There are better choices of sampling than proportionate stratified sampling to carry out this type of analysis. The disproportionate may be a better choice.
Disproportionate stratified sampling
Disproportionate sampling is a procedure in which the number of elements included in the sample from each stratum is not proportionate to their representation in the total population. The elements of the population do not have an equal chance of being included in the sample. The same sampling fraction does not apply to each stratum.
On the other hand, the strata have different sampling fractions, so this sampling procedure is not an equiprobable selection. The population composition must compensate for the sample’s disproportion to estimate the population parameters. However, for some research projects, disproportionate stratified sampling may be more appropriate than proportionate.
Disproportionate sampling can be divided into three subtypes based on the purposes of our assignment.
For example, it could facilitate strata analysis, focusing on optimizing cost, accuracy, or both accuracy and costs.
The objective of a study may require a researcher to carry out a detailed analysis of the sample strata. If proportionate stratification is used, the sample size of a stratum is very small; therefore, it may be challenging to meet the study’s goals.
The proportionate allocation may need to produce more cases for this type of detailed analysis. One option is to oversample small or infrequent strata. Such oversampling would create a disproportionate distribution of sample strata compared to the population. However, there may be sufficient cases to carry out the strata analysis required for the study.
LEARN ABOUT: Survey Sampling
Strengths and weaknesses of stratified sampling
Stratified sampling has many of the strengths and weaknesses associated with most probability sampling procedures compared to non-probability sampling procedures.
Compared to simple random sampling, the strengths of stratified sampling include the following:
- Ability to estimate population parameters and make inferences within each stratum and comparisons between strata. A simple random sampling method may need to capture more data on subgroups of interest. Stratified samples produce smaller random sampling errors than those obtained with simple random samples of the same size. A stratified sample will result in a sample that is at least as precise as a simple random sample of the same sample size.
- Stratified samples tend to be more representative of a population because it ensures that elements from each stratum in the population are represented in the sample. Sampling can be stratified to ensure the sample is spread over geographic subareas and population subgroups.
- Using stratified sampling, the researcher’s knowledge about the population is taken advantage of.
- Using stratified sampling allows the researcher to use different sampling procedures within different strata.
What is the difference between stratified sampling, sampling, and quota sampling?
Stratified sampling and quota sampling are similar to each other. Both involve dividing the target population into categories and then selecting a certain number of items from each category. Both procedures have as their main objective the selection of a representative sample and/or the facilitation of subgroup analysis. However, there are important differences.
The stratified random sampling method uses simple random sampling. A sampling process frame is needed for stratified sampling but not for quota sampling.
Advantages of quota sampling:
- It is the least expensive sampling method.
- It is widely used in polls and surveys by the media.
- The method assumes that the information we want to obtain is correlated with the population, but it is a hypothesis of representativeness that is difficult to prove.
In short, consider choosing stratified sampling if:
- Divining an entire population into two or more strata and building a homogeneous sampling frame for each stratum is possible.
- Some subgroups of the population are very different from other subgroups.
- It is essential to minimize sampling error.
- The population is heterogeneous.
- A comparative analysis of the strata is desired.
Finally, we share an article on the characteristics of another type of sampling, systematic sampling, cluster sampling.
Solve your research challenges with simple-to-create surveys that provide complex, real-time insights. Leverage custom point-and-click logic, advanced question types, and integrations and create mature, complex research models for choice-based, consumer, and more research.