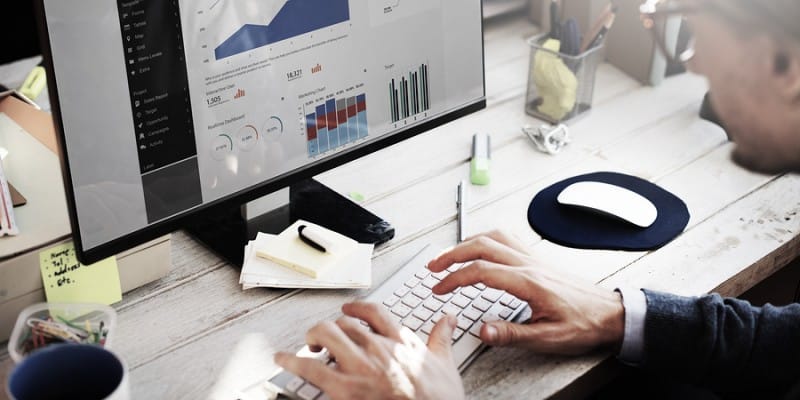
In marketing research, as well as other forms of social, economic and business research, metric data analysis (a.k.a interval and ratio data) is king. Yet as we have seen in other posts there is much that can be done with their lesser kin (nominal data and ordinal data). Our excitement as data analysts is piqued with interval and ratio data because they support a full range of statistical analysis tests and transformations.
There is a simple difference between interval and ratio data. Ratio data has a defined zero point. Income, height, weight, annual sales, market share, product defect rates, time to repurchase, unemployment rate, and crime rate are examples of ratio data where “zero” means just that.
LEARN MORE: Levels of Measurement: Nominal, Ordinal, Interval & Ratio
As an analyst you can say that a crime rate of 10% is twice that of 5% or annual sales of $2 million are 25% greater than $1.5 million. Ratio data can be transformed using logarithms, square roots, etc. to create ‘normal’ data, which is a requirement of certain statistical algorithms. In statistical analysis, distinguishing between categorical data and numerical data is essential, as categorical data involves distinct categories or labels, while numerical data consists of measurable quantities.
Interval data lacks an absolute zero point, which makes direct comparisons of a magnitude not possible (e.g. A is twice as large as B). We can utilize averages and standard deviations with interval data but again are limited in our ability to reference magnitude. Speaking of intervals, there is a seasoned debate regarding psychometric scales and whether or not they are interval or ordinal data. Purists argue they are ordinal, but many marketing researchers have accepted them as interval, thus allowing the use of multivariate statistics.
LEARN ABOUT: Market Evaluation
Survey tools offer several ways to capture interval and ratio data. For example, we can ask a respondent to state their income or in a B2B environment list a company’s annual sales. These figures can range from zero to infinity, which gives them ratio properties. Numerical data collected in this fashion can be categorized making it easier to create groups. For example, income collected in a survey in numerical fashion can be collapsed into equal groups (e.g. deciles, quartiles or terciles). Data collected in categorical fashion, however, cannot be broken out.
Platforms, such as QuestionPro, allow survey authors the ability to generate scales (e.g. Likert) where categories are assigned values from 1 – 5, 1 – 7, 1 – 9, etc. Given the argument listed above one can consider this data to be either ordinal or interval. A recent development is the use of scales that range from 0 – 10 or 0 to 100. These scales are commonly used to measure satisfaction, willingness to recommend (e.g. Net Promoter Score), or perceived economic conditions.
There are multiple question formats which can be used to generate interval and/or ratio data. This data is rich in potential for insight, but must be thought through carefully before deploying them on your survey.