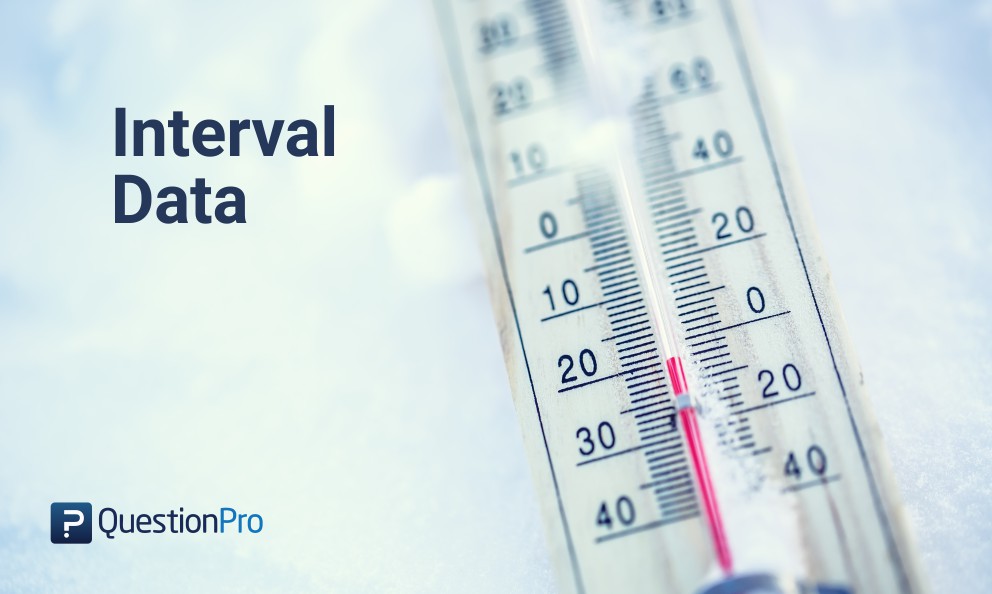
Interval Data: Definition?
Interval data, also called an integer, is defined as a data type which is measured along a scale, in which each point is placed at equal distance from one another. Interval data always appears in the form of numbers or numerical values where the distance between the two points is standardized and equal.
Interval data cannot be multiplied or divided, however, it can be added or subtracted. Interval data is measured on an interval scale. A simple example of interval data: The difference between 100 degrees Fahrenheit and 90 degrees Fahrenheit is the same as 60 degrees Fahrenheit and 70 degrees Fahrenheit.
In market research or in any other forms of social, economic or business research interval data plays a pivotal role. What makes interval data so popular and in-demand is because interval data supports almost all statistical test and transformations in obtaining quantitative data.
LEARN ABOUT: Test Market Demand
Interval data has very distinctive attributes that make it distinct in comparison to nominal data, ordinal data or even ratio data. Interval data doesn’t have a defined absolute zero point which is present in ratio data. The lack of absolute point zero makes comparisons of direct magnitudes impossible. For example, Object A is twice as large as Object B is not a possibility in interval data.
Learn more: Variable Measurement Scales- Nominal, Ordinal, Interval and Ratio.
Interval Data Analysis
Since interval data is quantitative analysis data type almost all the methods used to analyze quantitative can be used. Here are a few examples:
1. Trend analysis
Trend analysis is a popular interval data analysis technique, used to draw trends and insights by capturing survey data over a certain period of time. In other words, a trend analysis on interval data is conducted by capturing data using an interval scale survey in multiple iterations, using the same question.
2. SWOT Analysis
Analysis conducted to evaluate an organization’s strengths, weaknesses, opportunities, and threats is called SWOT analysis and is widely used to evaluate interval data. Strengths and weaknesses are internal aspects of an organization while opportunities and threat are external to an organization. An organization can measure interval data to evaluate market competition as well as plan future marketing activities using the SWOT analysis results.
LEARN ABOUT: Level of Analysis
3. Conjoint Analysis
Conjoint Analysis is an advanced level market research technique usually implemented to analyze how individuals make complicated decisions in an interval scale. Which factors are important for customers before they make decisions where they have multiple options available at their disposal.
4. TURF Analysis
TURF analysis stands for Totally Unduplicated Reach and Frequency analysis- is a method that allows a marketer to analyze the potential of market research for a combination of products and services. It evaluates the interval data of customers reached by a particular source of communication and its frequency. This analysis technique is used by researchers to understand whether a new product or service will be well-received in the target market or not. This unit of analysis method was primarily used for designing media campaigns but has expanded to being used in product distribution and line analysis.
Collect and Analyze Interval Data with Surveys
Key Characteristics of Interval Data
Here are a few characteristics of Interval data:
- Measurement: Interval data is measured using an interval scale, which not only shows the order and direction but also shows the exact difference in the value. For example, the markings on a thermometer or a ruler are equidistant, in simpler words they measure the same distance between the two markings.
- Interval Difference: The distances between each value on interval data is equal. For example, the difference between 10 cm and 20 cms is the same as 20 cms and 30 cms.
- Calculation: In interval data, one can add or subtract values but cannot divide or multiply. Almost all statistical analysis are applicable when calculating interval data, mean, mode, median etc.
- Point Zero: Absolute zero point is arbitrary, which means a variable can be measured even if it has a negative value like temperature can be -10 below zero but height cannot be below zero.
Interval Data Examples
1. One can measure time during the day using a 12-hour clock, this is a good example of interval data. Time in a 12-hour format is a rotational measure that keeps restarting from zero at set periodicity. These numbers are on an interval scale as the distance between them is measurable and comparable. For example, the difference between 5 minutes and 10 minutes is the same as 15 minutes and 20 minutes in a 12-hour clock.
2. The temperature measured in Fahrenheit and Celsius but not in Kelvin. If you measure temperature in Fahrenheit and Celsius then it will be considered interval data as 0 is arbitrary. But in Kelvin, 0 is absolute. There can’t be a temperature below zero degrees in Kelvin.
3. When you calculate intelligence score in an IQ test. There is no zero point for IQ. According to psychological studies, a person cannot have zero intelligence, therefore in this example, zero is arbitrary. IQ is numeric data expressed in intervals using a fixed measurement scale.
4. Test scores of examination like SAT. Scores in SAT test are in the range of 200-800. The numbers from 0 to 200 are not used when they scale the raw score (number of questions answered correctly) to the section score. The reference point is not an absolute zero, thus, it qualifies to become interval data.
5. Age is also a variable that can be measured on an interval scale. For example if A is 15 years old and B is 20 years old, it not only clear than B is older than A, but B is elder to A by 5 years.
Interval data is one of the most used data types. Survey tools offer several ways to capture interval data. When a survey is deployed to a respondent, with a certain demographic question that asks respondents to state their income, these figures can range from zero to infinity!
For example:
Please state your annual income
- Below $40,000
- $40,000- $60,000
- $60,000- $80,000
- $80,000- $100,000
- Above $100,000
Numerical data collected in this manner can be can be categorized into groups, in the above mentioned examples groups can based on the respondents annual income. People falling under same income category.
LEARN ABOUT: Average Order Value
There are multiple survey question types which can be used to generate interval data. This data obtained is rich for insights but a researcher must think through carefully before deploying them in a survey.
Collect and Analyze Interval Data with Surveys